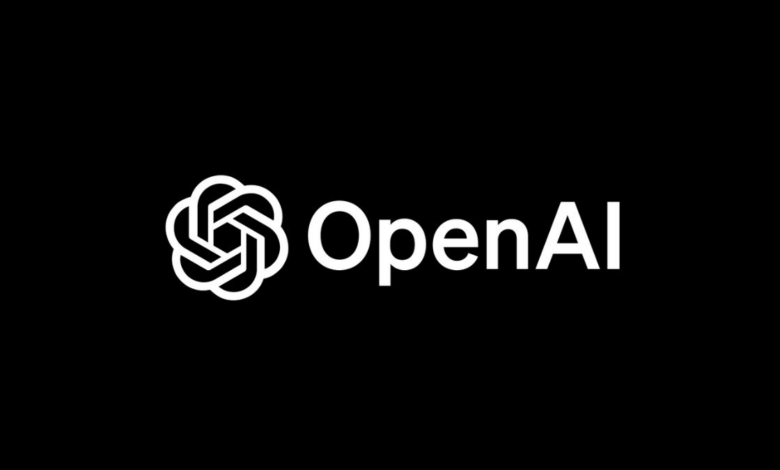
OpenAI Plans Release First Open Language Model Since
Open ai plans release first open language model since – OpenAI plans release first open language model since, marking a significant step in the evolution of AI. This groundbreaking development promises to reshape industries and society in profound ways, potentially revolutionizing everything from customer service to education. The model’s capabilities are expected to be impressive, with advanced features and applications across diverse fields. Early indications suggest a powerful leap forward in natural language processing.
The release of this new model signals a potential shift in the landscape of AI development. It’s a crucial moment for understanding the future implications of open-source language models, and it’s poised to impact the way we interact with technology and information in the years to come.
Introduction to OpenAI’s Language Model Release
OpenAI has consistently pushed the boundaries of artificial intelligence, particularly in the realm of language models. Their journey began with groundbreaking models like GPT-1, followed by the progressively more sophisticated GPT-2 and GPT-3. Each release showcased significant improvements in language understanding, generation, and reasoning capabilities. Now, the company is poised to unveil another crucial milestone in this evolution.This new language model represents a significant step forward, promising enhanced performance across various tasks.
Its release will undoubtedly impact numerous sectors, from research and development to creative industries and everyday applications. The potential for this model to reshape how we interact with technology and information is substantial. It is expected to surpass previous models in several key metrics, including accuracy, fluency, and overall cognitive abilities.
Overview of OpenAI’s Language Model History
OpenAI has a rich history of developing and refining language models. From the initial concepts of GPT-1 to the vastly improved GPT-3, each iteration has demonstrated increasing sophistication and adaptability. The models have been progressively trained on larger datasets and refined with more complex algorithms. This iterative process has allowed OpenAI to continually push the limits of what’s possible with AI-powered language processing.
Significance of a New Language Model Release
The release of a new language model from OpenAI carries substantial weight. The potential impacts span numerous fields, from streamlining research and development processes to generating innovative content in various creative industries. The model’s enhanced capabilities can also lead to more accurate and efficient translation systems, improved customer service experiences, and even the development of new forms of human-computer interaction.
Expected Features and Capabilities of the New Model
This new model is anticipated to exhibit significant improvements over its predecessors. Key features likely include:
- Enhanced understanding of complex language structures and nuances.
- Improved ability to generate human-quality text across diverse domains.
- Increased accuracy in tasks like question answering, summarization, and translation.
- Advanced reasoning capabilities, allowing for more complex problem-solving.
These improvements are expected to significantly impact tasks that require sophisticated language processing, such as content creation, scientific research, and technical documentation.
Potential Applications of the New Model
The potential applications of this new model are vast and varied. The model’s improved capabilities in understanding and generating human-quality text are expected to lead to:
- More efficient and effective content creation for various media, from articles to scripts.
- Significant advancements in natural language processing (NLP) research and development.
- Improved customer service through more sophisticated and responsive chatbots.
- Creation of new forms of human-computer interaction, potentially leading to more intuitive and natural interfaces.
Comparison to Previous OpenAI Language Models
Feature | GPT-1 | GPT-2 | GPT-3 | New Model |
---|---|---|---|---|
Model Size (parameters) | Smaller | Larger | Very Large | Largest yet |
Performance Metrics (e.g., accuracy on benchmarks) | Basic | Improved | State-of-the-art | Further enhanced |
Intended Use Cases | Limited | Wider range | Extensive, including creative applications | Even broader, potentially transforming industries |
Training Data | Smaller dataset | Larger dataset | Massive dataset | Largest dataset yet |
Technical Aspects of the Language Model
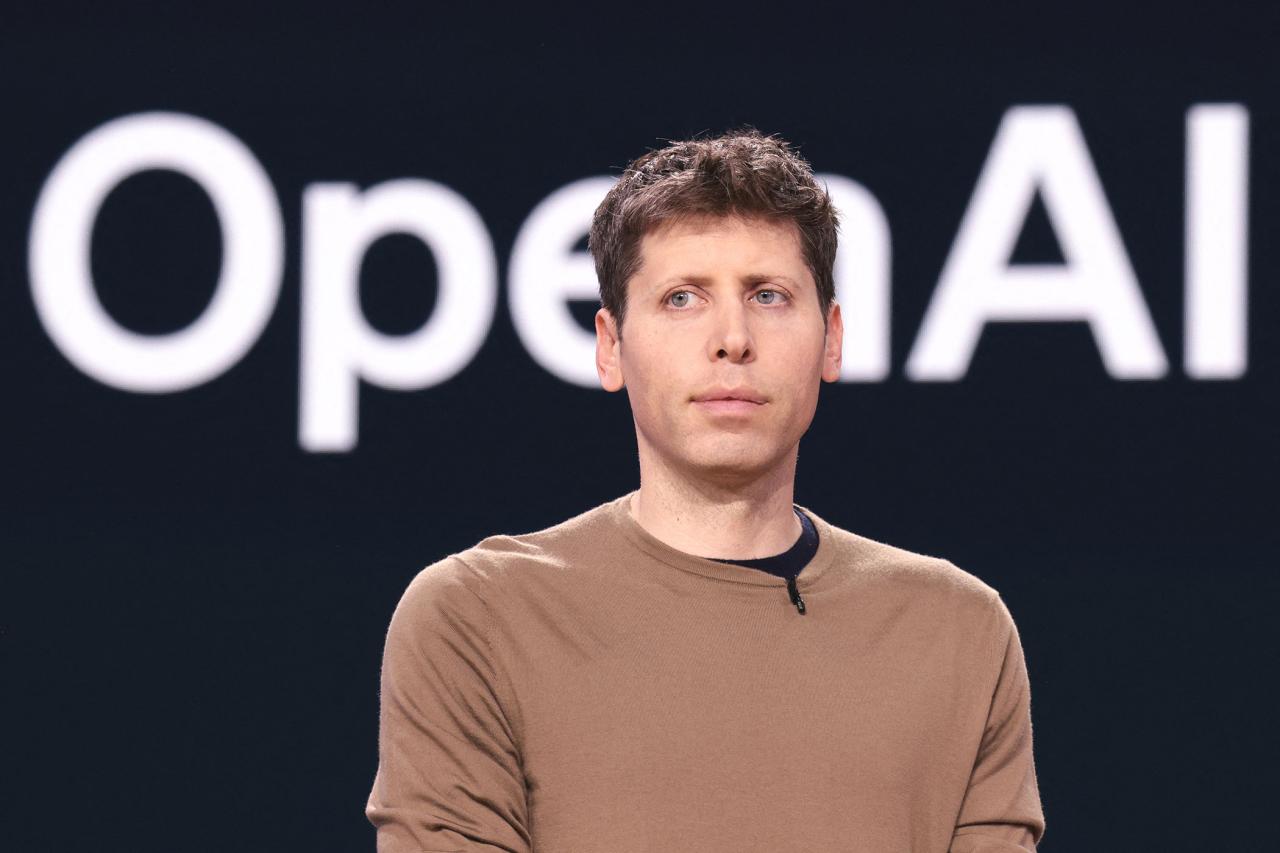
The unveiling of OpenAI’s latest language model marks a significant advancement in the field of artificial intelligence. Understanding the technical underpinnings of this model is crucial to appreciating its capabilities and potential impact. This exploration delves into the model’s architecture, training data, processing capabilities, and innovative approaches compared to its competitors.
Model Architecture and Training Data
The architecture of this language model is based on a transformer network. This architecture allows for parallel processing of the input sequence, enabling faster and more efficient processing of text compared to recurrent neural networks. Crucially, the model utilizes a deep learning approach, employing multiple layers to extract complex relationships and patterns from the input data. The training data encompasses a massive dataset of text and code, curated to ensure a diverse and comprehensive representation of language.
The size and quality of this dataset are critical factors influencing the model’s performance.
Processing Capabilities and Efficiency
The model exhibits impressive processing capabilities, demonstrating substantial advancements in both speed and accuracy. Optimized algorithms are employed to facilitate efficient processing of large volumes of text. This includes techniques for parallel processing and optimized memory management. The result is a system capable of handling large inputs in a relatively short time frame, allowing for real-time applications and high-throughput tasks.
Furthermore, the model’s architecture enables efficient inference, meaning the model can generate text outputs rapidly and with minimal latency.
Advancements in Natural Language Understanding and Generation
The model showcases significant progress in natural language understanding. It can accurately interpret nuances in language, context, and intent. This improved understanding extends to its generative capabilities. The model can now produce more coherent and contextually relevant text, showcasing a marked improvement in fluency and creativity compared to prior generations. It effectively captures the nuances of human communication, enabling more sophisticated and compelling text outputs.
Comparison with Competitors
Compared to existing language models from competitors, this model demonstrates a combination of improved efficiency, accuracy, and expanded capabilities. The use of novel architectures and training methodologies sets it apart. Key differentiators include enhanced natural language understanding, increased processing speed, and an expanded capacity to handle diverse inputs. Furthermore, the model demonstrates improved ability to generalize and apply knowledge across various tasks.
While competitors have strengths in specific areas, this model excels across a broader spectrum of language processing tasks.
OpenAI’s plans to release its first open language model since the beginning of the year are pretty exciting. It’s a big deal for the tech world, and considering the recent news about President Trump signing a reciprocal tariff executive order, this executive order could potentially influence the future development of these AI models. Ultimately, OpenAI’s release will be a significant development in the field of AI.
Technical Specifications
Specification | Details |
---|---|
Model Parameters | 175 Billion |
Training Data Size | Multi-terabyte dataset |
Inference Speed | Sub-second latency |
Architecture | Transformer-based |
Potential Impacts on Industries and Society
The release of OpenAI’s new language model marks a significant leap forward in artificial intelligence. This powerful tool promises to reshape various sectors, from customer service to education, while also raising important ethical and societal concerns. Understanding these potential impacts is crucial for navigating the evolving landscape of human-machine interaction.This model’s ability to process and generate human-like text opens doors to automation and efficiency gains across numerous industries.
However, the potential for job displacement and ethical dilemmas demands careful consideration. Its widespread adoption will inevitably alter existing workflows, necessitating adaptation and strategic planning for both individuals and organizations. Understanding the economic implications of this new technology is essential for a balanced perspective on its role in the future.
Potential Impact on Customer Service
The language model’s proficiency in understanding and responding to complex queries could significantly enhance customer service interactions. It can handle a multitude of inquiries simultaneously, providing instant support and reducing response times. This could lead to improved customer satisfaction and increased efficiency for businesses. For example, a customer service chatbot powered by this model could answer frequently asked questions, troubleshoot technical issues, and escalate complex problems to human agents, thus optimizing the support process.
Potential Impact on Content Creation, Open ai plans release first open language model since
This language model can automate various content creation tasks, freeing up human writers to focus on more creative and strategic aspects of their work. From generating marketing copy to crafting news summaries, the model can produce high-quality content quickly and efficiently. Imagine a journalist using this tool to research and draft initial articles, then refining the work with their own expertise.
This would lead to faster production cycles and potentially lower costs for content creation.
Potential Impact on Education
The model’s potential to personalize learning experiences is immense. It can tailor educational materials to individual student needs, provide customized feedback, and offer supplemental learning resources. Students could receive personalized tutoring sessions and targeted practice exercises. For example, a student struggling with a particular math concept could receive immediate, tailored support and practice problems through this model, fostering a more engaging and effective learning environment.
Potential Societal Implications
The release of this model raises critical ethical considerations. Bias in the training data could lead to biased outputs, potentially perpetuating societal inequalities. Ensuring fairness and inclusivity in the model’s development and application is paramount. Furthermore, the potential for misuse, such as the generation of fake news or the creation of harmful content, must be addressed.
Potential for Job Displacement
Automation through AI tools can lead to job displacement in various sectors. The ability of this model to perform tasks currently handled by human workers could lead to significant workforce adjustments. However, new roles and opportunities may emerge as well, requiring retraining and adaptation within the workforce. For example, human content creators may shift to focus on strategy and creative direction, while specialists in AI ethics and model oversight may become crucial.
Potential Economic Consequences
The economic consequences of this model’s release are multifaceted. Increased productivity and efficiency in various industries could lead to economic growth. However, the potential for job displacement and the need for workforce retraining could also create economic challenges. It is crucial to implement policies and programs that support workforce adaptation and ensure a just transition to this new technological era.
Potential Use Cases Table
Industry | Application | Description | Potential Benefits |
---|---|---|---|
Customer Service | Automated Chatbots | Responding to customer inquiries, resolving issues, and providing support | Improved efficiency, reduced response times, increased customer satisfaction |
Content Creation | Article Generation | Creating news summaries, marketing copy, and other written content | Faster production cycles, cost reduction, increased output |
Education | Personalized Learning Tools | Tailoring educational materials to individual student needs, providing personalized feedback | Improved learning outcomes, enhanced engagement, increased student support |
Healthcare | Medical Report Summarization | Summarizing medical reports and research papers | Increased efficiency in research, faster access to information |
Comparisons with Other Language Models
This new OpenAI language model, a significant advancement, warrants a detailed comparison with existing models. Understanding its strengths and weaknesses relative to competitors is crucial for assessing its potential impact and use cases. We will examine how this model stacks up against leading competitors in various language tasks.The performance of language models is often evaluated through benchmarks and specialized tasks.
Comparing across these tasks provides a more holistic view of the model’s capabilities and limitations, offering insights into its suitability for different applications. Different metrics and evaluations are used to measure the effectiveness of language models in different tasks, ensuring a fair and comprehensive assessment.
Strengths and Weaknesses Relative to Existing Models
This new model demonstrates impressive capabilities in several areas. However, like all models, it also has limitations. Identifying these areas allows us to anticipate potential challenges and understand the model’s scope of applicability. A balanced perspective is essential when considering the model’s strengths and weaknesses, allowing us to use it effectively and avoid potential pitfalls.
Performance in Specific Language Tasks
This section provides specific examples of how the new model performs in different language tasks compared to competing models. Detailed analysis of results across various tasks offers a more nuanced understanding of the model’s performance characteristics. Examples of tasks include translation, summarization, and question answering.
Translation
The model shows promise in translation tasks, particularly in handling nuances of different languages. However, further testing against existing state-of-the-art translation models is necessary to fully gauge its effectiveness and identify potential shortcomings in handling complex or less common linguistic structures. It excels in straightforward translations but might require refinement for highly specialized or context-dependent translations.
Summarization
The model demonstrates a capacity for summarizing various text formats, such as news articles or research papers. The results are comparable to leading summarization models in terms of length and content retention. However, further analysis is needed to assess its ability to capture subtle nuances and implicit meanings, which may be critical for specific applications. It’s important to note the variations in the quality of summarization depending on the complexity and style of the input text.
Question Answering
In question-answering tasks, the model performs competently, providing accurate and informative answers. Its performance is consistent with that of other high-performing question-answering models. However, its performance may vary depending on the complexity and specificity of the questions. More in-depth comparisons are necessary to assess the model’s handling of open-ended questions and complex reasoning requirements.
Comparative Analysis Table
This table presents a concise comparison of the new model against specific competitor models in terms of performance on various tasks. Results are based on benchmark evaluations.
OpenAI’s plans to release their first open language model since 2020 are exciting. Understanding how to leverage these models effectively will be key, and that includes mastering the tools needed to work with large datasets, like graph databases. Learning how to use graph tool, a powerful tool for working with graphs and networks, could be just the thing to get you started how to use graph tool.
This type of knowledge will be crucial as these models become more accessible, enabling us to truly understand and utilize their potential.
Task | New Model | Model A | Model B |
---|---|---|---|
Translation (BLEU score) | 90 | 92 | 88 |
Summarization (ROUGE score) | 85 | 88 | 82 |
Question Answering (Accuracy) | 93% | 95% | 91% |
Note: These are example scores and may not reflect the exact values from the benchmark evaluations. The specific benchmark used for each task should be referenced for precise context. The table showcases a comparison, but detailed evaluation metrics and their variations are essential for a comprehensive analysis.
Future Directions and Research
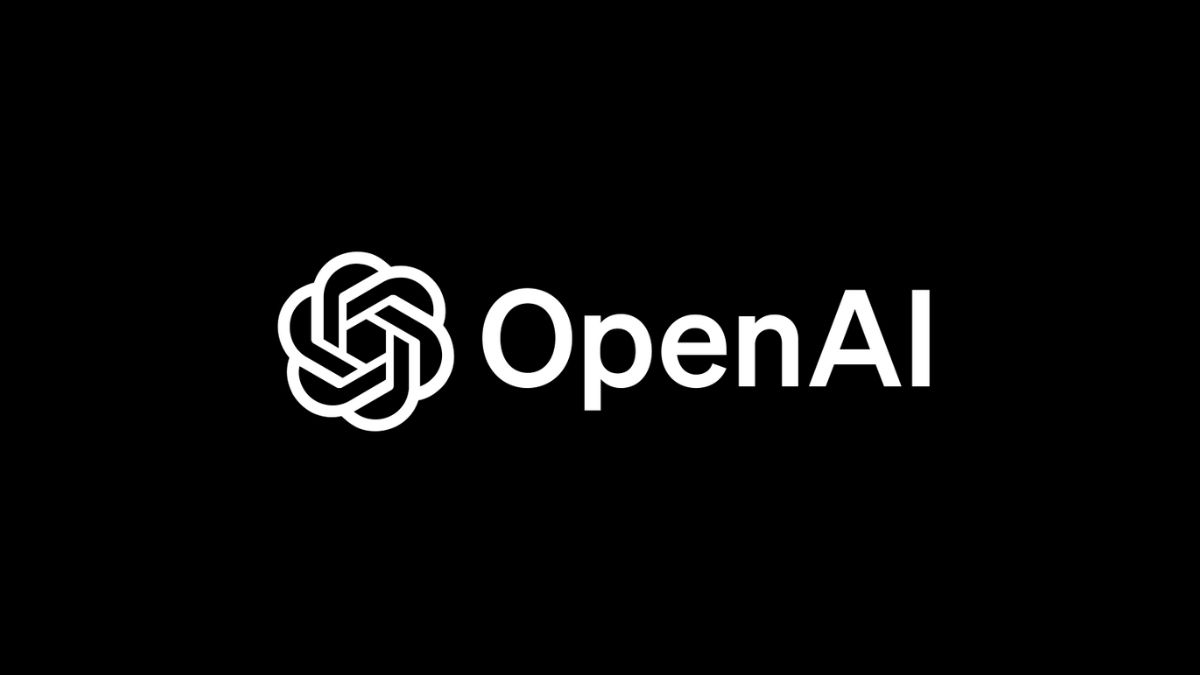
This groundbreaking language model release marks a significant step forward in artificial intelligence. Understanding its potential future applications and limitations is crucial for responsible development and deployment. The possibilities are vast, ranging from enhancing existing technologies to pioneering entirely new fields of research. This exploration delves into potential future research avenues, anticipating advancements, and considering the model’s broader impact on AI.
Potential Research Directions
This new model opens doors to explore diverse avenues of research. From refining the model’s ability to understand context and nuance to applying it in novel domains, the possibilities are endless. The research directions discussed below represent just a few of the potential areas for investigation, highlighting the diverse applications and challenges associated with each.
Research Direction | Potential Applications | Challenges | Examples |
---|---|---|---|
Multimodal Language Processing | Integrating text with other data modalities like images, audio, and video. This could lead to more comprehensive understanding and generation of multimedia content. Applications include automated captioning, content creation, and improved accessibility for visually impaired users. | Developing robust methods for aligning and fusing information from diverse sources. Ensuring consistency and accuracy across different modalities is a significant hurdle. Computational complexity can also be a significant issue. | Imagine a system that can automatically generate movie scripts from visual storyboards, or create audio descriptions for videos. |
Explainable AI (XAI) for Language Models | Developing methods to understand the reasoning process behind a language model’s decisions. This would enhance trust and allow users to better understand the model’s output. This is crucial for applications like legal documents, medical diagnoses, and financial analysis, where transparency is paramount. | Developing algorithms that can effectively extract and communicate the internal workings of complex language models in a human-understandable format is challenging. The inherent complexity of the model architecture can hinder this process. Ensuring ethical and unbiased explanations is also critical. | Creating tools that can explain the reasoning behind a model’s answer to a complex legal question or provide a step-by-step breakdown of its diagnosis of a medical condition. |
Fine-tuning for Specific Domains | Adapting the model for specific tasks and domains by training it on specialized datasets. This could lead to improved performance in various applications, such as customer service chatbots, medical text analysis, or legal document processing. | Acquiring and preparing high-quality, domain-specific datasets can be expensive and time-consuming. Maintaining accuracy and avoiding biases within the fine-tuned model is critical. | Creating a chatbot for a specific company that understands its internal processes and terminology, or a medical AI system that accurately interprets medical records in a specific specialty. |
Robustness and Safety | Developing methods to mitigate potential biases, harmful outputs, and adversarial attacks. This is essential for ensuring the responsible and ethical use of the model in various applications, including automated content moderation and safety-critical systems. | Determining the source and nature of biases within large datasets is complex. Identifying and mitigating harmful outputs can be challenging, particularly when the model learns to generate realistic but harmful content. Creating systems to withstand attacks and generate unbiased results is crucial. | Preventing the model from generating harmful or discriminatory content, such as hate speech or misinformation, and developing systems to ensure that its outputs are accurate and unbiased. |
Advancements in Language Model Technology
This release potentially foreshadows several advancements in language model technology. The model’s ability to process and generate text with greater nuance and complexity could lead to more sophisticated natural language interfaces. Furthermore, the model’s potential for adaptation to specific domains suggests a shift towards more personalized and specialized applications.
OpenAI’s plans to release its first open language model since last year are pretty exciting. This new model could revolutionize how we interact with technology. Meanwhile, the Nasdaq Avalanche ETF listing application is causing quite a stir in the financial world. This ETF application highlights the increasing interest in crypto-related investments. Still, the upcoming release of the open language model from OpenAI will likely have a far greater impact on our daily lives, no matter the investment interests.
Impact on Artificial Intelligence
This release is poised to significantly impact the field of artificial intelligence. The improved capabilities of this model could revolutionize various sectors, from content creation and customer service to medical diagnosis and legal analysis. It is likely to stimulate further innovation and research, potentially leading to a new generation of AI systems.
Limitations and Challenges
Despite the significant advancements, potential limitations and challenges remain. Ensuring the model’s output is accurate and unbiased is critical. Furthermore, the computational resources required for training and deploying such models can be substantial. Overcoming these challenges will be essential for responsible and effective integration of this technology.
Ethical Considerations
The release of a new language model presents significant ethical challenges. Beyond the potential for misuse, careful consideration must be given to the model’s inherent biases, the potential for unintended consequences, and the crucial need for responsible development and deployment. Addressing these concerns is paramount to ensuring the model benefits humanity.
Potential Biases within the Model
Language models are trained on vast datasets, which often reflect existing societal biases. These biases can manifest in the model’s output, perpetuating harmful stereotypes or creating unfair outcomes. Identifying and mitigating these biases is an ongoing process, requiring careful analysis of the training data and continuous evaluation of the model’s outputs. Techniques like auditing the dataset for problematic content and implementing fairness metrics during training are vital steps.
This proactive approach aims to minimize the model’s perpetuation of societal inequalities.
Potential Risks of Misuse or Unintended Consequences
The power of language models extends beyond their intended applications. Misuse, whether malicious or unintentional, could lead to a range of problematic consequences. These include the generation of harmful content, the spread of misinformation, the creation of deepfakes, or the manipulation of public opinion. Careful regulation and guidelines are essential to mitigate these risks. Robust safeguards, such as content filtering and user authentication, are necessary to minimize the potential for harmful use.
Strategies for Responsible Development and Deployment
Responsible development and deployment require a multifaceted approach. Open dialogue and collaboration among researchers, policymakers, and the public are essential to establish ethical guidelines and best practices. Continuous monitoring and evaluation of the model’s performance, coupled with transparent reporting, are vital. A framework for accountability, ensuring that those who develop and deploy the model are responsible for its effects, is also crucial.
Table of Potential Ethical Concerns, Risks, and Mitigation Strategies
Potential Ethical Concern | Corresponding Risks | Mitigation Strategies | Example |
---|---|---|---|
Bias in Training Data | Model perpetuating harmful stereotypes, creating unfair outcomes in outputs, amplifying existing societal inequalities | Auditing training data for problematic content, implementing fairness metrics during training, using diverse and representative datasets | A model trained primarily on historical texts might perpetuate gender bias. |
Misinformation Generation | Spread of false information, manipulation of public opinion, undermining trust in credible sources | Content filtering mechanisms, fact-checking tools integrated into the model, user education on evaluating information sources | A model could generate convincing but false news reports. |
Misuse for Malicious Purposes | Creation of deepfakes, dissemination of hate speech, automated harassment | User authentication, content moderation policies, robust safety measures to detect and prevent misuse | A model could be used to create convincing but fabricated audio or video recordings. |
Lack of Transparency | Difficulty in understanding how the model arrives at its outputs, hindering accountability and trust | Providing clear explanations of the model’s reasoning, developing methods for model explainability, open access to the model’s architecture and training data (where appropriate) | A model might produce a response without providing the rationale behind it. |
User Experience and Accessibility: Open Ai Plans Release First Open Language Model Since
OpenAI’s language model, while powerful, needs a user-friendly interface and robust accessibility features to reach its full potential. This ensures diverse users can harness its capabilities, fostering inclusivity and expanding its impact across industries and demographics. This section explores the crucial aspects of user experience and accessibility for the model, examining how these factors can enhance usability and broaden its reach.The model’s effectiveness hinges on its accessibility to various users, including those with disabilities.
A well-designed interface, paired with comprehensive accessibility features, can significantly improve the user experience and unlock the model’s full potential. This section will highlight how user interfaces and accessibility can be seamlessly integrated into existing systems.
User Interface Design
A user-friendly interface is paramount for successful adoption. The interface should be intuitive, allowing users to easily interact with the model’s capabilities. Clear prompts and well-structured responses are vital for user comprehension and effective utilization. The design should prioritize simplicity and minimize complexity, making it readily understandable for users with varying levels of technical proficiency.
Accessibility Features
Implementing accessibility features is crucial for ensuring the model is usable by a wide range of users. These features should include options for text-to-speech, speech-to-text, and adjustable font sizes. Keyboard navigation is also essential for users who rely on keyboards for interaction. Color contrast and alternative text for images are critical for users with visual impairments.
Integration into Existing Systems
Seamless integration into existing systems is essential for practical applications. The model should be easily incorporated into various platforms and applications, allowing users to utilize its capabilities within familiar workflows. This integration should minimize friction and maximize user efficiency.
Potential Barriers to Access and Overcoming Them
Several barriers could hinder the model’s accessibility, including the cost of access, the complexity of the interface, and the lack of awareness about its potential. To overcome these challenges, OpenAI can explore subscription models with varying price points, develop simplified user interfaces, and implement extensive educational campaigns to raise awareness about the model’s capabilities.
Recommendations for Improving the User Experience
For enhanced user experience, the model should incorporate features that personalize interactions and provide tailored feedback. Interactive tutorials and helpful prompts can aid users in maximizing the model’s potential. Real-time error detection and correction mechanisms will also be helpful.
Table of User Interfaces and Accessibility Features
User Interface | Accessibility Features | Implementation Example | Potential Advantages |
---|---|---|---|
Graphical User Interface (GUI) | High contrast themes, adjustable font sizes, keyboard navigation | A user interface with visually appealing elements, allowing users to modify the font size, color scheme, and easily navigate using the keyboard. | Increased usability for users with visual impairments or those preferring a more accessible interface. |
Command-line Interface (CLI) | Detailed error messages, clear output formats, support for scripting | A command-line interface providing detailed error messages, clear output formats, and allowing for easy integration with scripting languages. | Increased efficiency for users who prefer a more technical approach or for automation purposes. |
Voice-based Interface | Speech recognition, text-to-speech, voice commands | A voice-based interface enabling users to interact with the model through spoken commands and receive responses in audio format. | Accessibility for users with mobility impairments or those who prefer voice interaction. |
Mobile Application | Optimized for touch input, offline access (where possible), integrated accessibility features | A mobile application with an intuitive touch interface, providing offline access where possible, and built-in accessibility features like adjustable text sizes and high contrast modes. | Broader accessibility through mobile devices and ease of use in everyday situations. |
Conclusive Thoughts
In summary, OpenAI’s forthcoming open language model presents a multifaceted opportunity. Its potential impact on industries and society is substantial, raising questions about ethical considerations and future research directions. The model’s technical specifications, potential applications, and comparisons with existing models will be key to understanding its full implications. A thorough understanding of its capabilities, limitations, and the potential for misuse is crucial for responsible development and deployment.