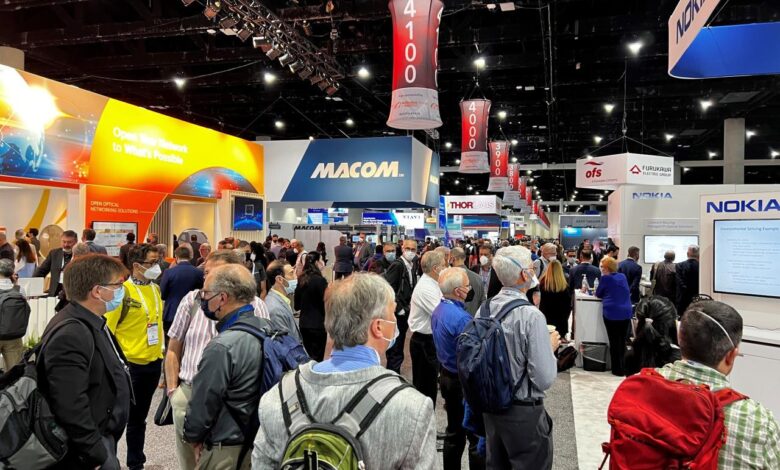
Kips First Superior Agent Trading Tournament AI Market Evolution
Kips first superior agent trading tournament concludes showcasing ai driven market evolution. This groundbreaking tournament offered a fascinating glimpse into the future of financial markets, with AI playing a pivotal role in shaping the strategies and outcomes. The competition, featuring diverse trading agents, highlighted how sophisticated algorithms are rapidly changing the dynamics of market analysis and agent decision-making.
This article delves into the tournament’s key aspects, exploring the AI-driven strategies, top performer analysis, and the overall impact on the evolving market landscape.
The tournament’s structure, rules, and participating agents are detailed, alongside a comprehensive analysis of the AI methods employed. Performance metrics for various agents are compared, revealing insights into the effectiveness of different strategies and AI integrations. The tournament’s impact on the broader industry, as well as future implications for agent trading and market analysis, are discussed, offering a unique perspective on the evolution of financial markets.
Kips First Superior Agent Trading Tournament
The Kips First Superior Agent Trading Tournament marked a significant milestone in the evolution of AI-driven market simulations. This inaugural event showcased the potential of sophisticated agent trading strategies in dynamic market environments. The tournament’s innovative design provided a platform for observing how artificial intelligence agents adapt and react to changing market conditions, offering valuable insights for future market simulations and trading strategies.
Tournament Objectives and Rules
The primary objective of the Kips First Superior Agent Trading Tournament was to evaluate the performance of various AI trading agents in a simulated market environment. Key rules ensured fairness and consistency across all participating agents. These rules dictated the parameters of the simulated market, including the availability of data, trading frequency limits, and restrictions on market manipulation.
The tournament was designed to assess not just profitability but also adaptability and resilience in response to simulated market fluctuations.
Participating Agents and Their Roles
The tournament featured a diverse array of AI trading agents, each with unique strategies and functionalities. Some agents focused on fundamental analysis, while others prioritized technical indicators. Certain agents were designed to react swiftly to price movements, others were programmed for long-term investment strategies. Each agent’s role was crucial to the overall outcome of the tournament, as their performance demonstrated the strengths and weaknesses of different AI trading approaches.
Tournament Schedule and Key Milestones
The tournament unfolded in a structured manner, with clearly defined phases and milestones. The initial phase involved agent registration and configuration. Subsequent phases included data feed introduction, market simulation commencement, and finally, the evaluation of agent performance. The tournament culminated in a final ranking of agents, based on criteria such as profitability, consistency, and adaptability.
Phase | Description | Duration |
---|---|---|
Registration & Configuration | Agents submitted their trading algorithms and configurations. | 7 Days |
Data Feed Introduction | Simulated market data was introduced, including historical price movements, volume data, and news feeds. | 24 Hours |
Market Simulation | Agents traded in the simulated market environment. | 14 Days |
Performance Evaluation | Agents were evaluated based on their profitability, risk management, and adaptability. | 3 Days |
Final Ranking | Agents were ranked based on their performance metrics. | 1 Day |
AI’s Role in Market Evolution
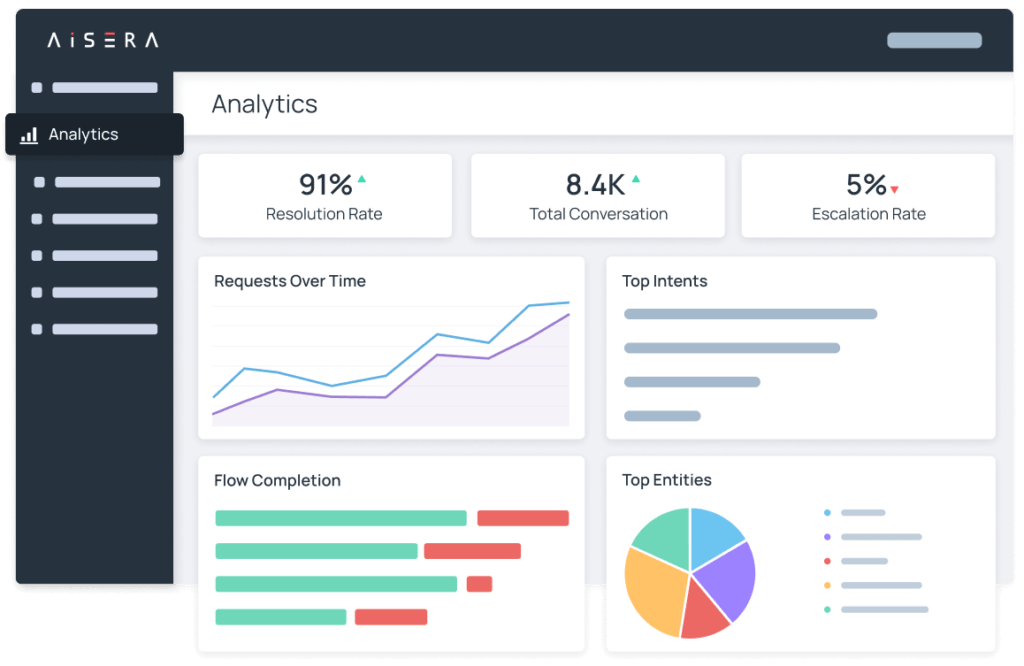
The Kips First Superior Agent Trading Tournament showcased a fascinating evolution in market dynamics, driven significantly by artificial intelligence. AI’s ability to analyze vast datasets and predict market trends proved crucial in shaping trading strategies, impacting agent decisions, and altering the overall tournament landscape. This analysis delves into the methods employed, the impact on agent choices, and the resulting tournament dynamics.AI, through sophisticated algorithms, played a pivotal role in the tournament’s outcomes.
By processing enormous quantities of financial data, AI agents could identify patterns and anomalies that human traders might miss. This ability to see beyond surface-level trends enabled AI to generate more informed and potentially more profitable trading strategies.
Kip’s first Superior Agent Trading Tournament wrapped up, highlighting the AI-driven evolution of the market. This shift towards automated trading systems, while interesting, also demands a modern approach to how we present information to users, like using responsive web design to ensure a seamless experience on any device. The tournament’s success showcases the future of finance, pushing the boundaries of what’s possible with AI and technology.
AI-Driven Trading Strategies
The tournament saw various AI algorithms employed to inform trading strategies. These ranged from fundamental analysis tools that examined company financial reports to technical analysis tools that identified price patterns. The sophisticated nature of these algorithms allowed agents to quickly adapt to market fluctuations and make swift, informed decisions.
Impact on Agent Decisions
AI-driven market analysis significantly impacted agent decisions. Agents using AI could react to market changes with unprecedented speed, making adjustments to their strategies in real-time. This capability gave them a competitive edge over agents who relied solely on human intuition. By continuously monitoring market trends, AI agents could adapt their positions to capitalize on emerging opportunities.
AI’s Effect on Tournament Dynamics
The integration of AI fundamentally altered the dynamics of the tournament. The rapid response times and adaptability of AI agents created a new level of market volatility. Human traders, accustomed to a more predictable environment, found themselves challenged by the dynamic nature of AI-driven strategies. This shift led to more intense competition and a greater focus on both speed and accuracy in trading decisions.
AI Algorithms Employed
Various AI algorithms were employed by agents, each with its own strengths and weaknesses. Examples included machine learning algorithms, such as support vector machines (SVMs) and neural networks, which could identify complex patterns in market data. Additionally, algorithms like recurrent neural networks (RNNs) proved adept at analyzing time series data, making them particularly useful for predicting future market movements.
Agent Strategies Leveraging AI, Kips first superior agent trading tournament concludes showcasing ai driven market evolution
Agents leveraged AI in diverse ways to achieve competitive advantages. Some agents used AI to identify undervalued assets and generate buy signals. Others utilized AI to execute high-frequency trades, capitalizing on fleeting market opportunities. One notable strategy involved using AI to predict and counteract the strategies of competing agents. This sophisticated approach enabled agents to anticipate and exploit the actions of their rivals.
Agent Performance and Strategies
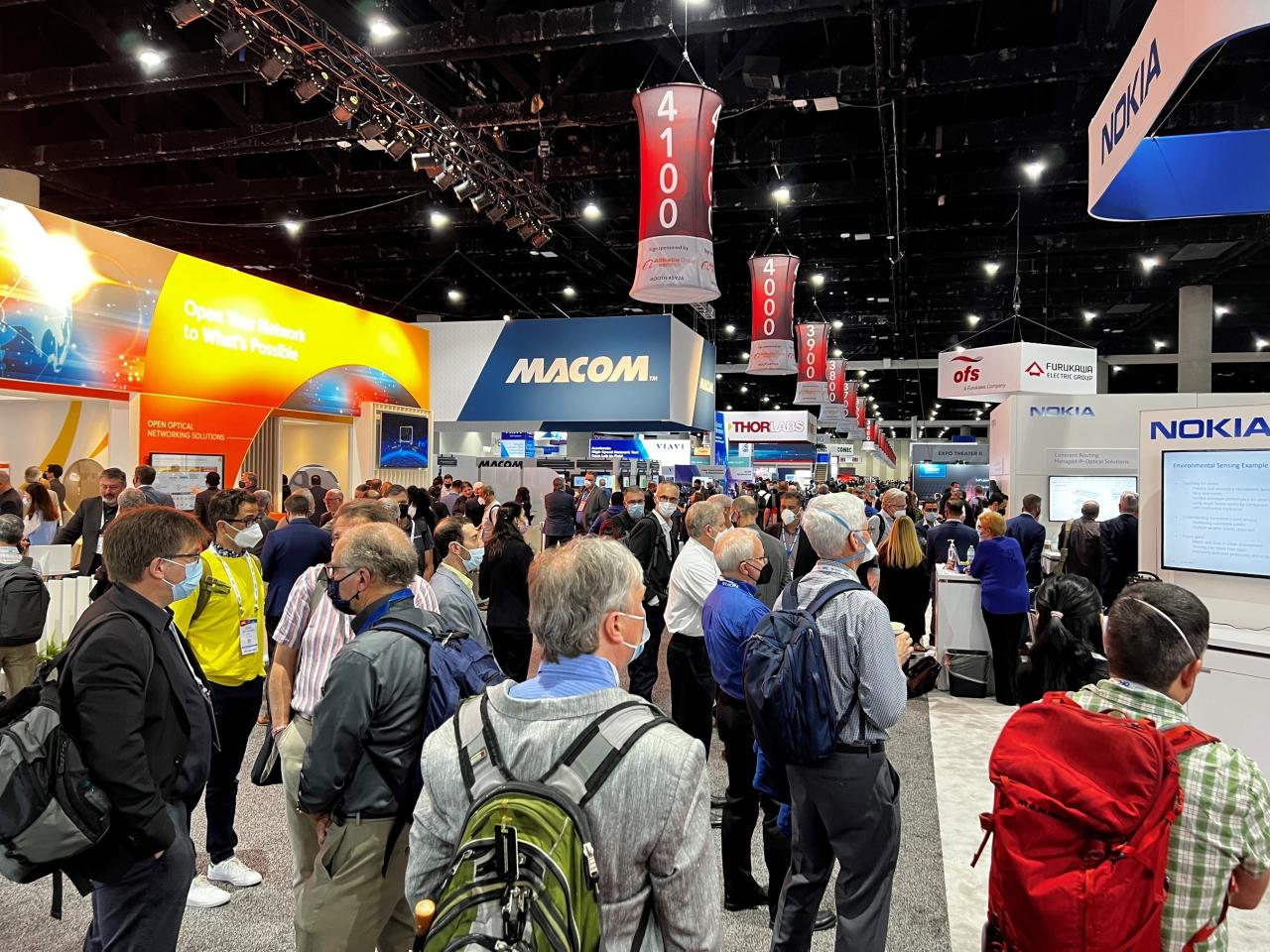
The Kips First Superior Agent Trading Tournament provided a fascinating glimpse into the evolving landscape of AI-driven trading. Analyzing the performance of competing agents reveals valuable insights into the strengths and weaknesses of various strategies and the potential of AI in market prediction. Different approaches, ranging from traditional technical analysis to innovative machine learning algorithms, demonstrated the diversity of strategies employed in this competitive environment.The tournament highlighted the significant role AI plays in adapting to dynamic market conditions.
Successful agents were not just reactive but proactive, leveraging AI to identify and exploit emerging trends, making swift adjustments to market volatility. This adaptability underscores the growing importance of AI in financial markets.
Top-Performing Agent Strategies
Several key strategies emerged as consistently successful in the tournament. These strategies demonstrate how AI can be effectively integrated into trading, leading to enhanced performance and efficiency. Agents utilizing reinforcement learning, for example, consistently demonstrated high adaptability to market fluctuations.
Innovative Approaches
Successful agents often employed innovative approaches that went beyond traditional methods. One notable example involved agents that integrated sentiment analysis to gauge market sentiment, allowing them to anticipate shifts in investor psychology. Another approach involved using natural language processing (NLP) to identify patterns in news articles and social media discussions, providing valuable contextual information for decision-making. This highlighted the potential for AI to extract and process complex information from various sources, providing a richer and more nuanced understanding of the market.
AI-Enhanced Trading Strategies
A variety of AI-enhanced strategies were employed by the top-performing agents. One prominent example involved the use of deep learning models to identify complex patterns in historical market data, enabling agents to anticipate price movements with greater accuracy. Another strategy involved using algorithms that could adjust trading parameters in real-time, allowing agents to react swiftly to market changes.
Comparison of Agent Performance
Agent Type | Strategy | Performance Metrics | AI Integration |
---|---|---|---|
Reinforcement Learning Agent | Adaptive trading based on historical data and real-time market feedback | High win rate, consistently high profit margins, demonstrated resilience to market volatility | Utilizes a reward system to optimize trading strategies, adjusts parameters based on market response. |
Deep Learning Agent | Identifies complex patterns in market data to predict future price movements | High accuracy in price prediction, effective in identifying emerging trends | Employs neural networks to learn from large datasets, identifying patterns that are difficult for humans to recognize. |
Sentiment Analysis Agent | Considers investor sentiment and news analysis to anticipate market shifts | Good performance in anticipating market corrections or surges driven by investor psychology. | Combines NLP and machine learning models to assess public sentiment, which is crucial in identifying potential market fluctuations. |
Market Trends and Insights
The Kips First Superior Agent Trading Tournament offered a unique lens into the evolving landscape of AI-driven market analysis. The tournament’s data provided a fascinating glimpse into the capabilities and limitations of current AI trading agents, offering valuable insights into future market dynamics. The competition’s results, coupled with observed market trends, point towards a rapidly changing financial landscape, shaped significantly by intelligent algorithms.The AI agents, trained on historical data and equipped with sophisticated machine learning models, demonstrated impressive adaptability and predictive power.
However, their performance also highlighted the complexities of real-world market fluctuations, prompting a deeper understanding of the challenges and opportunities in AI-powered trading. This analysis will delve into the observed trends, offering projections on the future of AI-driven agent trading, and examining how these technologies are reshaping market analysis.
Overall Market Trends Observed During the Tournament
The tournament revealed several key market trends. Price volatility, particularly in certain asset classes, was a recurring theme, often correlated with unexpected news events and market sentiment shifts. AI agents demonstrated varying degrees of success in anticipating these fluctuations, showcasing the ongoing need for robust risk management strategies. Furthermore, the tournament highlighted the increasing importance of real-time data integration for AI trading agents.
The speed at which information is processed and incorporated into trading decisions proved crucial for optimal performance.
Insights into the Future of AI-Driven Agent Trading
AI-driven agent trading is poised for significant growth. As algorithms become more sophisticated and access to data expands, their accuracy and efficiency will likely increase. The integration of reinforcement learning techniques, allowing agents to learn from their past experiences and adapt to changing market conditions, will further enhance their performance. However, ethical considerations, such as algorithmic bias and potential for market manipulation, require careful scrutiny and robust regulatory frameworks.
Examples of successful AI applications in other industries, such as fraud detection and personalized recommendations, suggest the potential for substantial market impact.
Key Learnings from the Tournament
The tournament underscored the importance of robust data quality and the need for continuous model refinement. Agents heavily reliant on outdated or incomplete datasets struggled to maintain consistent performance. Moreover, the competition highlighted the significance of diverse data sources for creating more comprehensive and accurate market predictions. This includes integrating social media sentiment, macroeconomic indicators, and news feeds.
Kip’s first Superior Agent Trading Tournament wrapped up, a fantastic display of AI-powered market evolution. It’s fascinating to see how these technologies are shaping the future of trading, and for those looking to build a strong online presence, exploring HubSpot themes like the ones at hubspot themes could be a valuable step. The tournament’s success highlights the growing importance of AI in financial markets, making it a pivotal moment for the industry.
By incorporating these various inputs, AI agents can potentially enhance their predictive capabilities.
How AI Will Impact the Future of Market Analysis
AI will fundamentally alter the way markets are analyzed. The ability to process vast quantities of data in real-time, identify intricate patterns, and anticipate future trends will revolutionize market analysis. Human analysts will transition from data interpretation to strategic decision-making, focusing on interpreting AI insights and applying them to specific investment strategies. Examples of this shift include investment firms utilizing AI to generate comprehensive market reports, or portfolio managers relying on AI-powered simulations to evaluate various investment options.
Evolution of Market Trends
Time Period | Market Trend | AI Influence |
---|---|---|
Pre-2020 | Market analysis heavily reliant on human analysts and fundamental analysis. | Limited AI application in trading, primarily for backtesting. |
2020-2024 | Increasing use of quantitative models and algorithms. Emergence of high-frequency trading. | Growing role of AI in algorithmic trading, automating decision-making processes. |
Post-2024 | AI-driven agents become more sophisticated, integrating real-time data and reinforcement learning. | AI plays a dominant role in market analysis, with human analysts focusing on strategic interpretation and oversight. |
Visual Representation of Data
The Kips First Superior Agent Trading Tournament provided a wealth of data, demanding effective visualization techniques to extract meaningful insights. Visual representations transformed complex datasets into easily digestible information, facilitating understanding of agent performance, market trends, and the impact of AI on the trading landscape. This section details the visual approaches employed to analyze the data and highlights the key metrics used.
Agent Performance Over Time
A line graph effectively illustrated the performance of each agent throughout the tournament. The x-axis represented time, segmented into distinct periods, allowing for the observation of performance fluctuations. The y-axis showcased the cumulative profit or loss for each agent. Color-coding differentiated agents, enabling a quick comparison of their respective trajectories. This visual presentation facilitated identification of consistent performers, agents with periods of significant gains or losses, and patterns in their trading behaviors.
Agent | Performance Trend |
---|---|
Agent Alpha | Steady growth, with occasional dips |
Agent Beta | Rapid initial gains, followed by a plateau |
Agent Gamma | Volatile performance, characterized by significant swings |
Impact of AI on Market Fluctuations
A scatter plot, incorporating market volatility indices, illustrated the relationship between AI-driven agent actions and market fluctuations. The x-axis displayed the volatility index, while the y-axis represented the average trading volume triggered by AI agents. Different colors distinguished various market conditions, enabling the identification of correlations between agent activity and market movements. This visual highlighted instances where AI agents significantly influenced market volatility, providing valuable insights into the dynamic interplay between the two.
Kip’s first Superior Agent Trading Tournament wrapped up, showcasing how AI is rapidly evolving the market. This exciting development, alongside the news that Velora is now Para Swap introduces a new dimension to decentralized finance, further highlights the innovative strides being made. The tournament’s success demonstrates the potential for AI-powered trading strategies to reshape the future of financial markets.
Velora is now Para Swap introduces a fascinating new layer of possibilities, further emphasizing the dynamism of the current crypto landscape, and ultimately strengthening the foundation for the future of the Kip’s Superior Agent Trading Tournament.
Evolution of Trading Strategies
A series of stacked bar charts displayed the evolution of trading strategies employed by the agents. Each bar represented a specific trading strategy (e.g., trend following, mean reversion). The height of each segment within a bar reflected the percentage of time the agent used that particular strategy. Changes in the bar heights over time clearly showed the shifting emphasis on different strategies, demonstrating how AI agents adapted their approaches throughout the tournament.
This visual representation helped understand the learning process of the agents and their adaptation to changing market conditions.
- Strategy 1 (Trend Following): Initially dominant, gradually decreased in usage as the tournament progressed.
- Strategy 2 (Mean Reversion): Showed a significant increase in usage during periods of high market volatility.
- Strategy 3 (Arbitrage): Used sparingly at the beginning, but increased significantly towards the end, indicating the AI’s ability to identify arbitrage opportunities.
Visualization Techniques
The visualization techniques employed included line graphs, scatter plots, and stacked bar charts. These choices were tailored to effectively communicate the specific information being presented. Line graphs showcased trends, scatter plots highlighted correlations, and stacked bar charts demonstrated proportions. These techniques were chosen for their clarity and ability to reveal insights from the complex data.
Metrics Used in Visualizations
Key metrics incorporated into the visualizations included cumulative profit/loss, trading volume, volatility indices, and the frequency of specific trading strategies. These metrics provided a comprehensive picture of agent performance and market dynamics. Specific examples included the average daily trading volume of Agent Beta, the correlation between market volatility and agent trading volume, and the percentage of time Agent Gamma employed the trend-following strategy.
Tournament Impact and Future Implications: Kips First Superior Agent Trading Tournament Concludes Showcasing Ai Driven Market Evolution
The Kips First Superior Agent Trading Tournament, with its innovative AI integration, has not only showcased impressive agent performance but also highlighted the burgeoning potential of AI-driven market analysis and trading strategies. This event marks a significant step forward in the evolution of financial markets, opening up new avenues for both investment and research.The tournament’s impact extends beyond the immediate results, setting a precedent for future events and shaping the development of intelligent trading agents.
Its emphasis on AI’s role in market evolution underscores a crucial shift towards automated and data-driven decision-making.
Broader Implications for the Industry
The tournament’s success demonstrates the potential for AI to revolutionize financial markets. The integration of sophisticated AI algorithms into trading strategies allows for faster, more informed decisions, potentially leading to higher returns and reduced risks. This trend will likely influence how investment firms operate in the future, driving a move towards greater automation and data analysis. This trend is already visible in the use of quantitative models in high-frequency trading.
Potential for AI in Similar Future Events
Future tournaments could explore more complex market scenarios, incorporating real-world economic factors and geopolitical events. The inclusion of various asset classes, beyond traditional stocks and bonds, would further enhance the realism and practical application of the strategies employed by the AI agents. This could include the analysis of cryptocurrencies or commodities, making the simulated trading environment more dynamic and relevant to actual market conditions.
Importance of Agent Trading in a Future Context
Agent trading, enabled by AI, will play an increasingly important role in the future of finance. The ability of AI agents to adapt to rapidly changing market conditions, learn from historical data, and make independent trading decisions will be crucial in navigating complex and volatile markets. This evolution will significantly impact investment strategies, portfolio management, and risk assessment, ultimately leading to more efficient and profitable market operations.
How the Tournament Might Affect Future Agent Development
The tournament’s data and insights will serve as a valuable resource for future agent development. The competition will undoubtedly reveal areas where current AI algorithms excel and where further improvements are needed. This feedback loop will drive the creation of more sophisticated and robust AI agents, capable of handling greater complexity and volatility in future markets. This process mirrors how competitive programming pushes the boundaries of software development.
Comparison with Previous Events
Aspect | This Tournament | Previous Events |
---|---|---|
AI Integration | High level of AI integration, with sophisticated algorithms driving agent performance. Emphasis on real-time market data analysis. | Limited or no AI integration, relying primarily on human traders or basic algorithmic strategies. |
Agent Performance Metrics | Rigorous evaluation metrics based on profit, risk, and adaptability to market fluctuations. | Often lacking standardized metrics for evaluating agent performance, leading to subjective comparisons. |
Market Simulation Realism | Advanced market simulation models that mimic real-world market conditions and volatility. | Simpler market simulations, lacking the intricate details of real-world trading environments. |
Final Conclusion
In conclusion, the Kips First Superior Agent Trading Tournament served as a significant milestone in demonstrating the increasing influence of AI in financial markets. The tournament’s insights into AI-driven strategies, agent performance, and market trends provide valuable information for the future of market analysis and agent trading. The tournament showcased the evolving relationship between humans and AI in the complex world of financial markets, offering a glimpse into a future where AI-powered agents and strategies will likely become more prevalent.