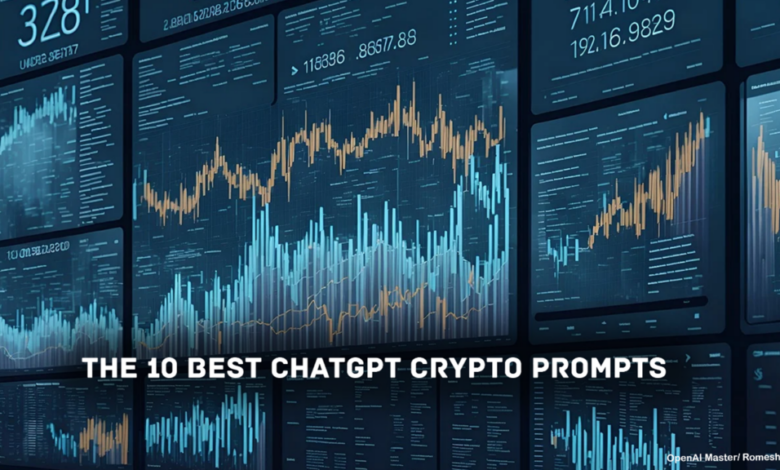
Predicting Crypto Trends with AI
How to use chatgpt to predict crypto market trends – How to use AI to predict crypto market trends opens up a fascinating new frontier in investment strategies. The volatile nature of the crypto market makes accurate prediction a continuous challenge. This guide explores the use of advanced analytical methods to potentially uncover hidden patterns and anticipate market movements, although no method guarantees success.
We’ll delve into data analysis techniques, model building, and interpretation, covering everything from sourcing and pre-processing data to understanding the inherent limitations of any prediction model. We’ll also examine real-world case studies, risk management strategies, and the future potential of this exciting intersection of AI and finance.
Introduction to Crypto Market Prediction
The cryptocurrency market is notoriously volatile, with prices fluctuating dramatically in short periods. This inherent volatility makes predicting future trends a significant challenge. Factors like regulatory changes, technological advancements, and overall market sentiment can all influence price movements, making precise forecasts difficult. While no method guarantees success, understanding the various approaches and their limitations can empower investors to make more informed decisions.The key to navigating this volatility lies in employing data analysis to identify potential patterns and trends.
By examining historical data, market indicators, and other relevant information, investors can develop hypotheses about future price movements. However, it’s crucial to acknowledge that predictive models are not foolproof. Their accuracy is always contingent on the quality and relevance of the data used and the assumptions embedded within the models.
Data Analysis in Crypto Prediction
Data analysis plays a crucial role in informing crypto market predictions. By examining historical price data, trading volume, and social media sentiment, analysts can identify potential patterns and correlations. This data-driven approach can offer valuable insights into market dynamics, though the complexity of the crypto market and the frequent emergence of new factors can limit the effectiveness of such analyses.
Potential Benefits and Limitations of Predictive Models
Predictive models can offer insights into potential future price movements, potentially helping investors make more informed decisions. For example, identifying a potential trend towards increased adoption of a particular cryptocurrency might lead to a strategic investment decision. However, it’s important to acknowledge the limitations. No model can perfectly predict the future, and external factors can easily disrupt any predictions.
Furthermore, models are only as good as the data they are trained on. Inaccurate or incomplete data can lead to inaccurate predictions.
Different Methods of Predicting Crypto Trends
Understanding the various approaches to predicting crypto trends can provide investors with a more comprehensive perspective. Each method has its strengths and weaknesses, and the most effective strategy often involves combining multiple approaches.
Method | Description | Strengths | Weaknesses |
---|---|---|---|
Technical Analysis | Identifies patterns and trends in price charts and trading volume to predict future price movements. | Relatively easy to understand and implement. Can identify short-term price fluctuations. | Does not account for underlying factors. Can be prone to overfitting and lagging indicators. |
Fundamental Analysis | Evaluates the intrinsic value of a cryptocurrency based on factors like the project’s team, technology, and market adoption. | Provides a long-term perspective. Considers the underlying value of the cryptocurrency. | Can be subjective and difficult to quantify. Requires significant research and analysis. |
Sentiment Analysis | Examines social media discussions, news articles, and other sources to gauge public sentiment towards a cryptocurrency. | Can identify emerging trends and shifts in public opinion. Provides a real-time perspective on market sentiment. | Subjective interpretation is required. Can be influenced by news events or misinformation. |
Machine Learning | Utilizes algorithms to identify patterns in data and predict future outcomes. | Can analyze large datasets and identify complex relationships. Potentially more accurate than other methods. | Requires significant computational resources and expertise. Model performance depends heavily on data quality. |
Data Sources for Crypto Prediction
Predicting crypto market trends is a complex endeavor, requiring a deep understanding of the underlying factors. Access to reliable and comprehensive data is crucial for developing accurate models and informed strategies. This section explores the various public datasets relevant to crypto markets, detailing how to gather and preprocess them, highlighting the significance of data quality, and discussing potential biases.Understanding the different sources of data available is the first step toward creating robust predictive models.
Different data points reflect different aspects of the market, from price fluctuations and trading volumes to news sentiment and social media chatter. Each data source provides a unique perspective, which, when combined, can offer a more holistic view of the market’s dynamics. Proper data preprocessing and handling are equally crucial, as raw data often contains inconsistencies and errors that can significantly affect the accuracy of predictions.
Figuring out crypto market trends with ChatGPT is fascinating, but visualizing those predictions can make them even clearer. Learning how to use motion graphics templates, like those available at how to use motion graphics template , can help you present your ChatGPT-generated insights in a dynamic and engaging way. This can help you present your analysis more effectively, whether you’re pitching an investment strategy or simply sharing your findings with friends.
Ultimately, combining ChatGPT’s predictive power with visually appealing motion graphics is a great way to communicate your crypto market insights.
Public Datasets
A variety of publicly available datasets offer insights into crypto market activity. These include historical price data, trading volume information, and news sentiment analyses. Each dataset type provides a unique perspective on the market.
Gathering and Preprocessing Data
Gathering data involves extracting information from various sources. This often requires using APIs, web scraping techniques, or downloading data directly from dedicated platforms. After collection, the data must be meticulously cleaned and preprocessed. This often includes handling missing values, transforming data types, and addressing inconsistencies. For example, converting date formats, handling different data units (like currencies), and removing outliers can significantly improve the quality of the data.
“Data preprocessing is a crucial step in ensuring the reliability and accuracy of any predictive model.”
Data Quality and Potential Biases, How to use chatgpt to predict crypto market trends
Data quality is paramount in crypto market prediction. Inconsistent data, missing values, and errors can lead to inaccurate models and unreliable predictions. Data quality can be affected by factors such as data entry errors, inconsistencies in reporting standards, and the frequency of data updates. Understanding potential biases is equally important. Data from specific exchanges or regions might not represent the entire market, and the way data is collected can introduce bias.
For instance, news sentiment analysis might be skewed toward certain news outlets or social media platforms. Carefully evaluating the sources and potential biases is essential to build unbiased models.
Using ChatGPT to predict crypto market trends can be surprisingly helpful, but remember it’s not a crystal ball. You can input historical data and ask for potential future price movements, but always treat the results as a starting point for your own research. This kind of data analysis is useful for any business, including local business marketing strategies.
For instance, analyzing customer sentiment and competitor activity using ChatGPT insights can help you fine-tune your marketing strategies. Ultimately, leveraging ChatGPT for crypto market prediction requires a blend of AI-driven insights and a healthy dose of common sense. local business marketing strategies can benefit from this same kind of data-driven approach, making it a valuable tool across various sectors.
Data Source Overview
Data Source | Strengths | Weaknesses |
---|---|---|
CoinMarketCap | Comprehensive market data, including prices, market capitalization, and trading volume. Real-time updates. | May not be fully accurate in reflecting all exchanges, data may be skewed by popular exchanges. |
CoinGecko | Similar to CoinMarketCap, with extensive data on various cryptocurrencies. Offers APIs for easy data access. | Similar limitations to CoinMarketCap regarding potential exchange bias. |
CryptoCompare | Extensive data on trading volumes, historical price data, and order books from various exchanges. Provides a more detailed picture of market activity. | Can be more expensive to access than free alternatives. May not cover all exchanges. |
News APIs (e.g., Reuters, Bloomberg) | Provides real-time news sentiment data, enabling analysis of market reactions to events. | Requires careful filtering and processing to remove irrelevant or biased news, often requires paid subscriptions. |
Social Media APIs (e.g., Twitter) | Provides insights into public sentiment towards cryptocurrencies through social media discussions. | Public sentiment can be influenced by influencers or bots, making analysis challenging. Requires significant data processing to isolate genuine sentiment. |
Methods for Analyzing Crypto Data
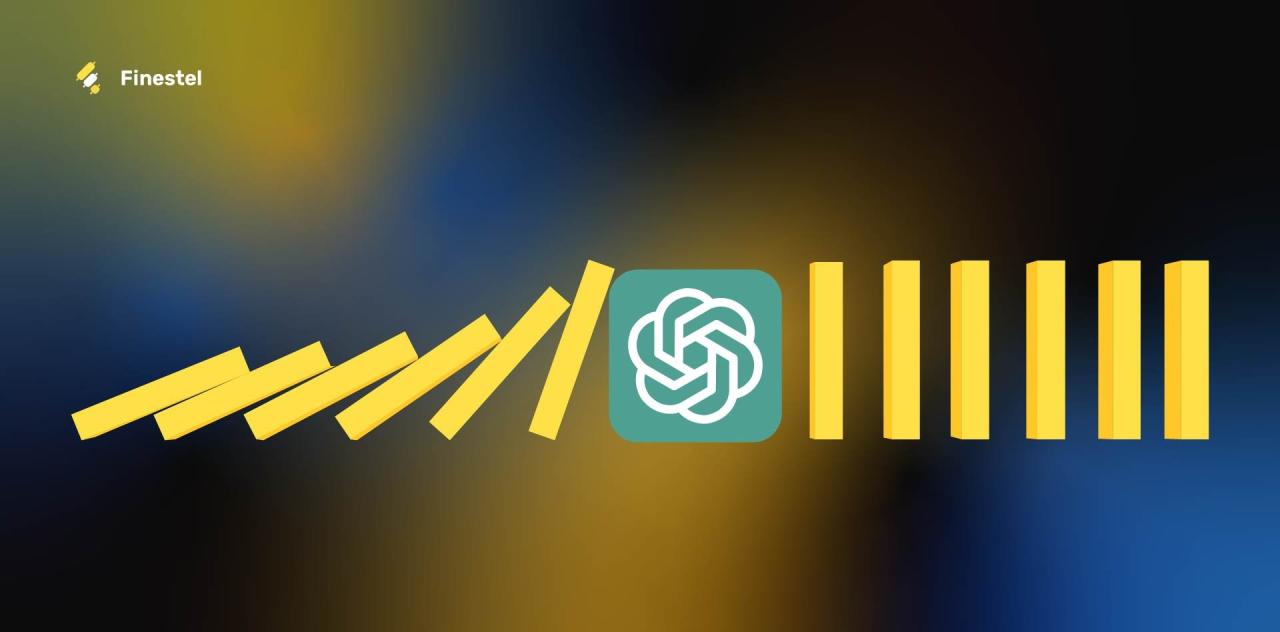
Predicting crypto market trends requires a deep dive into the data. Simply looking at price charts isn’t enough. Sophisticated methods are needed to uncover patterns and potential future movements. This section explores various techniques, from statistical modeling to machine learning, for analyzing crypto data and building predictive models.
Statistical Modeling Techniques
Statistical modeling provides a framework for understanding the underlying relationships within crypto market data. These techniques often involve identifying trends, seasonality, and correlations between different cryptocurrencies or market indicators.
- Time Series Analysis: This method focuses on analyzing historical price data to identify patterns and predict future price movements. Techniques like ARIMA (Autoregressive Integrated Moving Average) and Exponential Smoothing can be employed to model the time-dependent nature of crypto prices. For instance, ARIMA models can capture the cyclical nature of crypto market booms and busts.
- Regression Analysis: Regression models explore the relationship between a dependent variable (e.g., cryptocurrency price) and one or more independent variables (e.g., news sentiment, trading volume). Linear regression, for example, can help quantify the impact of specific factors on price fluctuations. If news sentiment consistently correlates with price changes, this analysis could highlight that link.
- Correlation Analysis: This technique investigates the relationships between different variables. Understanding how different cryptocurrencies or market indicators correlate can provide insights into potential interconnectedness. For instance, a strong positive correlation between Bitcoin and Ethereum prices might suggest a similar market sentiment.
Machine Learning Algorithms
Machine learning offers a powerful toolkit for predicting crypto market trends by identifying complex patterns and relationships within large datasets.
- Support Vector Machines (SVM): SVMs are effective for classification tasks, potentially predicting whether a cryptocurrency’s price will rise or fall. The algorithm identifies optimal boundaries between different price categories.
- Decision Trees and Random Forests: These models can analyze various factors influencing price movements and build decision rules to predict future trends. For instance, a decision tree might consider volume, volatility, and market sentiment to classify price movements.
- Neural Networks: Neural networks, particularly deep learning models, excel at handling large, complex datasets. These models can identify intricate patterns and relationships in crypto market data, leading to more accurate predictions. Deep learning models are capable of identifying subtle, non-linear relationships in the data that might be missed by other methods.
Steps in Building a Predictive Model
Building a predictive model involves several key steps:
- Data Collection and Preparation: Gathering relevant historical data from reliable sources is critical. This includes price data, trading volume, and potentially other relevant indicators. Cleaning the data to handle missing values and outliers is crucial.
- Feature Engineering: Creating new features from existing data can improve model performance. This might include calculating technical indicators, volume-weighted averages, or market sentiment scores.
- Model Selection and Training: Choosing the appropriate algorithm for the task, and then training it on the prepared data. This involves optimizing model parameters to achieve the best possible accuracy.
- Model Evaluation: Assessing the model’s performance on unseen data to gauge its predictive power. This often involves splitting the dataset into training and testing sets.
- Deployment and Monitoring: Implementing the model into a system to provide real-time predictions and continuously monitoring its performance. Re-training the model with new data periodically is important.
Common Pitfalls in Data Analysis
Several pitfalls can undermine the accuracy and reliability of crypto market predictions.
- Overfitting: A model that performs exceptionally well on the training data but poorly on unseen data. This occurs when the model learns the noise in the training data rather than the underlying patterns.
- Data Bias: Data collected might reflect a specific period or market condition, leading to inaccurate predictions in different contexts. This bias must be considered.
- Lack of Feature Selection: Including irrelevant or redundant features in the model can decrease performance. Careful selection of features that genuinely influence the target variable is essential.
- Ignoring External Factors: Market predictions must incorporate macroeconomic factors, regulatory changes, and social trends.
Building a Predictive Model: How To Use Chatgpt To Predict Crypto Market Trends
Predicting crypto market trends is a complex endeavor, requiring careful consideration of various factors and sophisticated models. Building a robust predictive model involves selecting appropriate algorithms, preparing the data meticulously, and rigorously evaluating the model’s performance. This process demands a deep understanding of the crypto market dynamics and the limitations of any predictive tool.Constructing a predictive model is an iterative process.
It requires experimentation with different approaches and a commitment to refining the model based on performance evaluation. The goal is to create a model that provides meaningful insights into potential future price movements, while acknowledging the inherent uncertainty in the crypto market.
Model Selection
Choosing the right algorithm is crucial for accurate predictions. Various machine learning models, such as regression, classification, and time series analysis, can be applied to crypto data. The selection depends on the specific nature of the data and the desired outcome. For example, a regression model might be suitable for predicting price based on technical indicators, while a time series model could capture the cyclical patterns inherent in crypto markets.
The choice should be guided by factors like the nature of the data, the desired level of complexity, and the computational resources available.
Data Preparation
Data preparation is often a time-consuming but essential step in building a predictive model. Raw data needs to be cleaned, transformed, and preprocessed before it can be used for training. This includes handling missing values, outliers, and ensuring data consistency. Crucially, features relevant to price prediction need to be extracted from the raw data. This could involve calculating technical indicators like moving averages, relative strength index (RSI), or volume.
Want to use ChatGPT to predict crypto market trends? It’s all about sifting through data and patterns. Think about how you can leverage ChatGPT’s analytical abilities to find hidden connections. To make your insights even more compelling, incorporating visual elements like those in video email marketing could greatly enhance your communication. Presenting your ChatGPT-powered predictions in a dynamic, engaging format will undoubtedly grab attention, just like well-crafted video emails, and potentially yield more successful results in conveying your crypto market insights.
The goal is to create a dataset that is both complete and relevant for training the model.
Model Training
Training the model involves feeding the prepared data into the chosen algorithm. This process adjusts the model’s parameters to minimize errors in predicting future prices. The training data is typically divided into training and validation sets to assess the model’s performance on unseen data. The model is then trained iteratively, with adjustments to its parameters, aiming for the lowest possible error on the validation set.
Model Validation
Model validation is crucial for assessing the model’s predictive accuracy and generalizability. Common metrics for evaluating model performance include Mean Absolute Error (MAE), Root Mean Squared Error (RMSE), and R-squared. These metrics provide insights into the model’s ability to predict future prices accurately. A model with a low MAE and RMSE indicates better predictive accuracy, while a high R-squared suggests a stronger relationship between the model’s predictions and the actual data.
Model Retraining and Updating
The crypto market is dynamic and volatile. Therefore, models need periodic retraining and updating to reflect the changing market conditions. This involves acquiring new data, retraining the model, and evaluating its performance on the updated dataset. Regular updates are vital to maintaining the model’s accuracy and relevance. This iterative process ensures that the model continues to adapt to the ever-changing landscape of the crypto market.
Interpreting Prediction Results
Decoding the output of a predictive model for crypto market trends requires careful analysis. Simply seeing a number or a graph isn’t enough. Interpreting the results involves understanding the model’s strengths and weaknesses, the context of the data used, and the inherent uncertainties in forecasting the volatile crypto market. A thorough understanding of the model’s output allows traders to formulate informed decisions, recognizing that predictions are just one piece of the puzzle.Understanding the nuances of the model’s predictions is crucial.
It’s not about blindly following the output but rather understanding the probability distributions behind the predictions. For example, a model might predict a 70% chance of a price increase. This doesn’t mean it’s guaranteed, but it offers valuable context for risk assessment and portfolio management. Interpreting these probabilities requires a keen eye for the potential pitfalls of the prediction.
Quantifying Uncertainty in Predictions
Accurate prediction of crypto market trends is challenging due to the unpredictable nature of the market. Model outputs often include confidence intervals or prediction ranges. These intervals provide a measure of the uncertainty surrounding the predicted values. A wider range indicates higher uncertainty, while a narrower range suggests greater confidence in the prediction.For example, if a model predicts a price between $20,000 and $25,000 for Bitcoin in the next quarter, with a 95% confidence interval, it means that there’s a 95% chance that the actual price will fall within this range.
The remaining 5% represents the potential for the price to deviate significantly from the predicted range. It’s important to remember that even a high confidence interval doesn’t guarantee accuracy.
Translating Model Outputs into Actionable Insights
Predictive models provide valuable data, but translating this data into actionable trading strategies requires a thoughtful approach. Consider the following factors when formulating strategies based on predictions:
- Risk Tolerance: The level of risk a trader is comfortable with should heavily influence the trading decisions based on predictions. A prediction with a high degree of uncertainty might be inappropriate for a risk-averse trader. Conversely, a prediction with a lower degree of uncertainty may be suitable for a trader with a higher tolerance for risk.
- Market Context: The overall market sentiment and other relevant economic factors should be considered. A prediction in a bullish market might be more impactful than in a bearish one. It’s crucial to analyze the current market environment and compare it to the predicted conditions.
- Model Limitations: Predictive models are not perfect. Understanding the model’s limitations and biases is vital. For example, if the model was trained on data from a specific period, it might not accurately reflect the current market conditions.
Limitations of Relying Solely on Predictions
While predictive models can be valuable tools for understanding potential market trends, it’s crucial to remember their limitations. Predictions should never be the sole basis for trading decisions. They should be combined with fundamental analysis, technical analysis, and other factors that influence market behavior.For instance, a model might predict a significant price increase in a particular cryptocurrency. However, this prediction doesn’t account for external factors, such as regulatory changes, major news events, or shifts in investor sentiment.
A comprehensive trading strategy should incorporate these external influences to create a more robust approach to the market.
Case Studies of Crypto Market Prediction
Predicting the crypto market is a notoriously difficult task, a wild ride filled with exhilarating highs and crushing lows. While no method guarantees success, analyzing past attempts can offer valuable insights into the factors that influence predictions and the strategies that have worked (or haven’t). Examining case studies, both successful and unsuccessful, provides a crucial lens through which to understand the complexities of this volatile market.Understanding the successes and failures of past predictions helps to refine our approach and develop more robust strategies for the future.
This is especially crucial given the unique characteristics of the crypto market, such as its decentralized nature, the rapid pace of innovation, and the significant influence of speculation.
Successful Prediction Attempts
Predicting price movements in the crypto market is a challenging endeavor. Some attempts, though not guaranteed, have shown promising results. Often, these successful predictions hinge on a combination of fundamental analysis (examining the project’s utility and team), technical analysis (identifying patterns and trends in price charts), and macroeconomic factors (global economic conditions).
- Predicting the surge in Bitcoin prices following a major news event, such as a positive regulatory development or a significant adoption by a prominent company, can be considered a successful example. These predictions often involve a thorough understanding of the market’s sentiment and the impact of specific events.
- Successful predictions also often rely on the identification of specific patterns in the price charts. These patterns, while not always definitive, can provide hints of potential future price movements.
Unsuccessful Prediction Attempts
Many prediction attempts have fallen short of their targets. These failures often stem from a misunderstanding of the market’s inherent volatility, the impact of unforeseen events, and the influence of speculation. One critical factor is the difficulty in accurately predicting the influence of external factors, like regulatory changes or unexpected technological advancements.
- A common example of an unsuccessful prediction involves predicting a price surge that never materialized due to unforeseen market sentiment shifts. This highlights the significant role of investor psychology in influencing the market.
- Predicting the timing and magnitude of price corrections, or downturns, can also be challenging. Factors such as market sentiment and the release of crucial information can dramatically influence the timing and depth of a correction.
Comparison of Prediction Strategies
Different approaches to crypto market prediction have varying degrees of success. The combination of technical analysis, fundamental analysis, and sentiment analysis plays a crucial role.
Prediction Strategy | Description | Success Rate | Lessons Learned |
---|---|---|---|
Fundamental Analysis | Examining the project’s utility, team, and market potential. | Moderate | While valuable, fundamental analysis alone often proves insufficient to predict price movements accurately. |
Technical Analysis | Identifying patterns and trends in price charts. | Variable | Technical analysis can provide insights, but its effectiveness is highly context-dependent. |
Sentiment Analysis | Analyzing social media sentiment and news articles to gauge market mood. | Moderate | Sentiment can influence price movements, but requires careful calibration and interpretation. |
Factors Contributing to Success or Failure
The success or failure of a crypto market prediction hinges on numerous factors. These factors include the accuracy of the underlying data, the effectiveness of the prediction model, the impact of external factors, and the interpretation of results.
- Data quality is paramount. Inaccurate or incomplete data can lead to flawed predictions.
- The sophistication of the prediction model plays a vital role in the outcome.
- Unforeseen external events, such as regulatory changes or major news announcements, can significantly impact price movements.
Risk Management and Trading Strategies
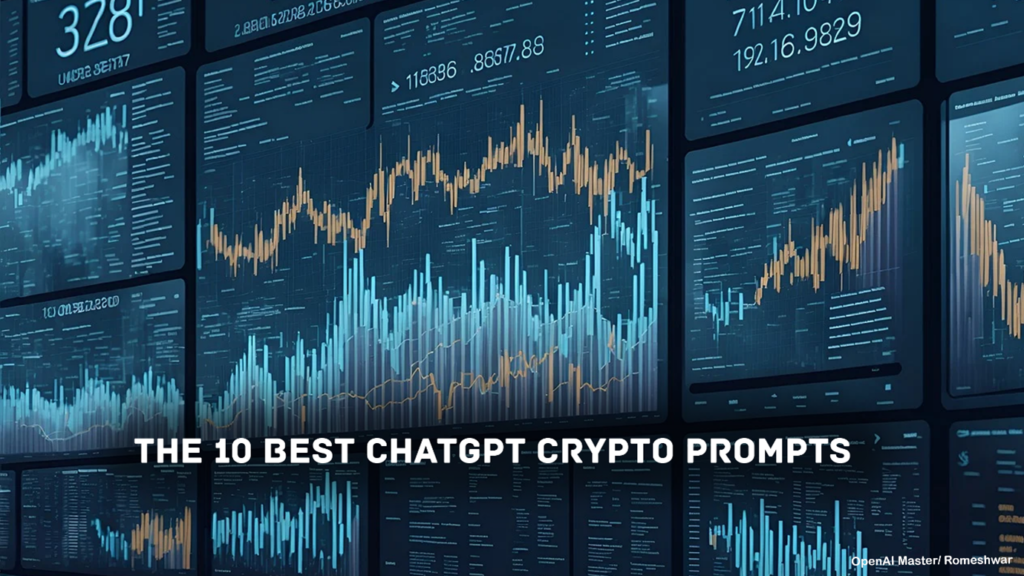
Cryptocurrency trading, while potentially lucrative, carries significant risks. Unpredictable market fluctuations and the inherent volatility of digital assets demand a robust risk management approach. Integrating sound trading strategies with accurate predictions is crucial for mitigating potential losses and maximizing profits.
Importance of Risk Management
Effective risk management in cryptocurrency trading is paramount. It’s not just about protecting capital; it’s about preserving the long-term viability of a trading strategy. Ignoring risk can lead to substantial losses, potentially eroding your entire investment. Understanding and quantifying potential risks allows traders to make informed decisions and avoid catastrophic outcomes.
Strategies for Incorporating Predictions into Trading Decisions
Successful crypto trading hinges on the ability to use predictions effectively. Predictions are valuable tools but should never be the sole basis for trading decisions. Combining predictions with technical analysis, fundamental research, and your own risk tolerance is crucial. For example, if a prediction suggests a price increase, you might look for indicators like volume and price action to confirm the signal before entering a position.
Setting Stop-Loss Orders and Profit Targets
Stop-loss orders and profit targets are fundamental tools in risk management. A stop-loss order automatically sells a position when the price reaches a predetermined level, limiting potential losses. Profit targets, conversely, automatically sell a position when the price reaches a desired profit level, securing gains. Properly setting these levels is essential, balancing potential reward with the acceptable level of risk.
For example, a stop-loss order set at 10% below the current price can safeguard your investment if the market moves unexpectedly against you.
Trading Strategies and Associated Risks
Different trading strategies cater to varying risk tolerances and investment goals. A crucial aspect of any strategy is the risk assessment. Here’s a table outlining some common strategies and their inherent risks:
Trading Strategy | Description | Associated Risks |
---|---|---|
Day Trading | Involves holding positions for short periods (typically a day or less). | High risk of substantial losses due to rapid price fluctuations. Requires significant market knowledge and quick decision-making. |
Swing Trading | Involves holding positions for a few days to a few weeks. | Moderately high risk compared to long-term holding. Requires a good understanding of market trends and price action. |
Long-Term Holding | Involves holding positions for months or years. | Lower risk compared to other strategies but may miss out on short-term gains. Requires strong conviction in the underlying asset. |
Scalping | Involves making numerous small profits in a short period. | High risk, requiring significant technical expertise and rapid response to market movements. |
Careful consideration of your individual risk tolerance and market conditions is crucial before employing any strategy. Each strategy has inherent risks that need to be managed diligently.
Future Directions and Advancements
Predicting crypto market trends is a complex task, constantly evolving with the development of new technologies and market dynamics. Improving predictive models requires continuous innovation and adaptation to the ever-changing landscape of cryptocurrency. This section explores potential avenues for advancement, focusing on areas ripe for research and development, and the role of AI and machine learning in enhancing prediction accuracy.
Improving Predictive Model Accuracy
Future advancements in crypto market prediction hinge on the development of more sophisticated models that can account for intricate market nuances and incorporate real-time data. Current models often struggle with the volatility and unpredictability of crypto markets, necessitating refinements to improve their predictive capabilities. Techniques like incorporating sentiment analysis of social media data and news articles, along with more sophisticated algorithms for handling high-frequency trading data, will likely lead to more accurate and robust predictions.
Enhancing Data Sources and Integration
The availability and quality of data significantly impact the reliability of predictions. Future research should focus on expanding data sources to encompass a wider range of market participants and transaction types. For instance, incorporating data from decentralized exchanges (DEXs) and smaller altcoins could provide a more comprehensive view of the market. Furthermore, integrating data from various sources and formats, potentially using advanced data fusion techniques, will allow for a more holistic picture of market activity, ultimately leading to improved predictions.
Leveraging AI and Machine Learning
Artificial intelligence (AI) and machine learning (ML) offer promising avenues for enhancing predictive models. Deep learning models, capable of extracting complex patterns from massive datasets, can potentially uncover hidden correlations and predict future market movements with greater accuracy. Reinforcement learning algorithms can also be used to optimize trading strategies based on real-time market conditions. Advanced neural networks, specifically designed for high-frequency trading and complex market analysis, could significantly improve prediction accuracy and speed.
Addressing Limitations and Ethical Considerations
Despite the potential of AI and ML, potential limitations exist. One key consideration is the potential for model bias, stemming from the data used to train the model. Ensuring data diversity and fairness in model training is crucial. Moreover, the inherent complexity of crypto markets can lead to model overfitting, where the model performs well on historical data but poorly on new data.
Developing robust techniques to mitigate overfitting and ensure generalizability is essential. Finally, the ethical implications of using AI for automated trading, particularly in the context of market manipulation, need careful consideration and regulation.
Case Studies and Real-World Applications
A few case studies demonstrate the potential of advanced models. For instance, a model incorporating sentiment analysis and on-chain data might have predicted the recent surge in Bitcoin’s price by detecting a surge in positive sentiment on social media and a surge in on-chain activity. This highlights the importance of incorporating diverse data sources.
Potential Future Trends
The future of crypto market prediction will likely involve the integration of multiple advanced techniques. This will include incorporating more comprehensive data sets, sophisticated AI models, and a deeper understanding of the underlying market mechanisms. By addressing the limitations and ethical concerns associated with these advancements, we can foster a more accurate and trustworthy approach to crypto market prediction.
Closing Summary
In conclusion, predicting crypto trends with AI is a complex endeavor that requires a multifaceted approach. While AI can uncover valuable insights, it’s crucial to remember the inherent volatility of the market and the importance of risk management. This guide provides a framework for understanding the process and empowers readers to make informed decisions in the ever-evolving crypto landscape.
Remember, no prediction is foolproof.