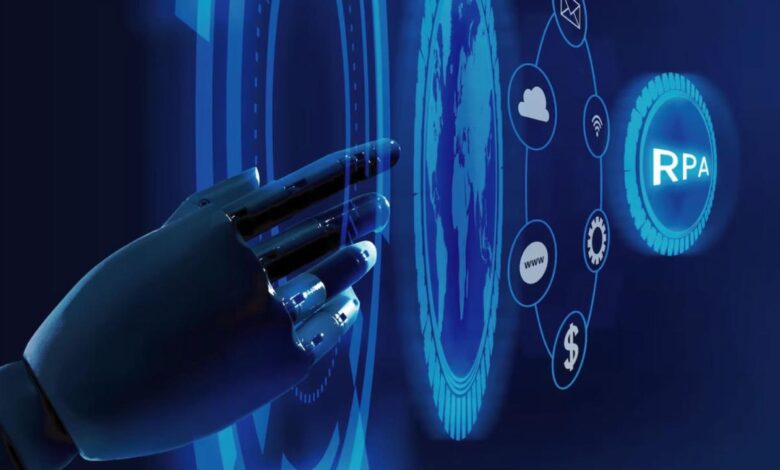
Core Scientific Data Center 1-2 Billion Coreweave AI
Core scientific data center 1 2 billion coreweave ai – Core scientific data center 1-2 billion coreweave AI promises a revolutionary approach to managing massive scientific datasets. Imagine a facility capable of storing and analyzing petabytes of data, powered by cutting-edge AI like Coreweave. This isn’t just about storage; it’s about unlocking new scientific discoveries through unprecedented data processing capabilities. This exploration delves into the intricacies of such a facility, from its defining characteristics to the innovative technologies driving its capabilities.
The core scientific data center’s design will address critical issues in data integrity, security, and scalability. The integration of Coreweave AI will be crucial in extracting insights from the vast datasets, while maintaining high throughput and low latency. A comprehensive overview of data management processes, security protocols, and future trends will complete this discussion.
Defining Core Scientific Data Center: Core Scientific Data Center 1 2 Billion Coreweave Ai
A core scientific data center is a specialized facility designed to support the unique needs of scientific research and discovery. It’s not just another data center; it’s a critical infrastructure component for handling, processing, and archiving the vast amounts of data generated by modern scientific instruments and experiments. Its purpose extends beyond simple storage; it involves the rigorous management of data integrity, security, and accessibility for the scientific community.These centers are more than repositories; they are dynamic hubs for collaborative research, enabling scientists to analyze, interpret, and draw insights from the data.
The careful design and implementation of these facilities are crucial for ensuring the reproducibility and validity of scientific findings. Data integrity and security are paramount, guaranteeing the long-term preservation and usability of valuable scientific information.
Purpose and Function, Core scientific data center 1 2 billion coreweave ai
A core scientific data center is a specialized facility designed for managing large-scale scientific datasets. It is equipped with advanced infrastructure, including high-performance computing resources, robust storage systems, and sophisticated data management tools. These centers facilitate the collection, storage, processing, and dissemination of data from diverse scientific disciplines. The key function is to provide a secure and accessible environment for scientists to conduct research and collaborate effectively.
They are instrumental in fostering interdisciplinary scientific advancements.
Characteristics Distinguishing a Core Scientific Data Center
These centers are distinguished from other data centers by several key characteristics. Firstly, their data is typically highly structured and complex, requiring specialized storage and processing solutions. Secondly, data integrity and security are paramount, often exceeding industry standards for commercial data centers. Thirdly, these facilities often support specific research projects or disciplines, with the data curated and managed according to the unique needs of that domain.
Fourthly, data accessibility and sharing are prioritized, often through open-source policies and standardized formats. Lastly, the technical expertise and specialized personnel required to manage and maintain these facilities are crucial to its success.
Role of Data Integrity and Security
Data integrity and security are fundamental pillars within a core scientific data center. The rigorous standards ensure the accuracy, reliability, and trustworthiness of scientific data. This involves implementing strict data validation procedures, redundancy measures, and access controls. Robust security protocols protect sensitive data from unauthorized access, alteration, or loss, crucial for preserving the integrity of scientific research.
Data backups and disaster recovery plans are essential to safeguard against potential data loss.
Types of Scientific Data
A core scientific data center handles diverse scientific data types. These include:
- High-throughput sequencing data from genomics research, often requiring massive storage capacity and specialized analysis tools.
- Astronomical data from telescopes, encompassing images, spectra, and other observational data.
- Climate data from weather stations and satellites, demanding consistent storage and sophisticated analytical capabilities.
- Experimental data from physics, chemistry, and materials science experiments, which often requires complex data formats and advanced computational tools.
The diverse types of data highlight the broad applications of core scientific data centers in various scientific disciplines.
Comparison of Core Scientific Data Center and Commercial Data Center
The following table illustrates the key differences between a core scientific data center and a commercial data center:
Feature | Core Scientific Data Center | Commercial Data Center |
---|---|---|
Data Type | Highly structured, complex scientific data | Diverse, unstructured data (e.g., customer transactions, web traffic) |
Data Integrity | High priority, adhering to strict scientific standards | Important, but less stringent compared to scientific data |
Data Security | Robust, often exceeding industry standards | Secure, but tailored to commercial needs |
Data Accessibility | Prioritized, often through open-source policies | Controlled, access based on user roles and permissions |
Data Management | Specialized, often domain-specific tools | General-purpose tools, less specialized |
Expertise | Highly specialized scientific personnel | Generally IT professionals |
The table demonstrates the unique focus and specialized requirements of a core scientific data center, setting it apart from a typical commercial data center.
The Core Scientific Data Center 1, boasting 2 billion Coreweave AI cores, is impressive, but the recent surge in South Korean crypto exchange users hitting 16 million here hints at a potential shift in how these powerful computational resources are being leveraged. It’s fascinating to consider how this data center might be influencing the future of the crypto market, and by extension, the global economy.
The sheer scale of the Coreweave AI project remains fascinating.
Coreweave AI Integration
The integration of Coreweave AI into a core scientific data center presents a transformative opportunity to accelerate research and discovery. Leveraging the power of artificial intelligence to analyze vast datasets will unlock insights that traditional methods might miss. This integration promises to be a crucial element in advancing scientific progress in numerous fields.Coreweave AI’s ability to process and interpret complex scientific data, combined with the high-throughput capabilities of the data center, will significantly improve the speed and efficiency of research.
The Core Scientific Data Center 1, with its 2 billion Coreweave AI processors, is pushing the boundaries of what’s possible in data analysis. This incredible computational power opens up fascinating avenues for generating realistic human voices, like those found in the ai voice generator. Ultimately, this powerful infrastructure in the Core Scientific Data Center 1, 2 billion Coreweave AI will be key to unlocking more advanced applications in the future.
However, careful consideration of potential challenges and limitations is essential for a successful implementation. Understanding the strengths and weaknesses of different AI models and their compatibility with the data center infrastructure is critical to maximize the return on investment.
Potential Benefits of Coreweave AI Integration
Leveraging Coreweave AI’s capabilities offers significant advantages in a scientific data center. Improved data analysis speed, leading to quicker insights and discoveries, is a key benefit. Automation of routine tasks, freeing up human researchers for more complex and creative work, is another important advantage. The ability to identify hidden patterns and correlations within large datasets, often missed by human analysis, leads to the potential for groundbreaking discoveries.
Ultimately, the integration enhances the overall productivity and efficiency of the scientific process.
Potential Challenges and Limitations
Implementing Coreweave AI into a core scientific data center presents several challenges. Ensuring the data quality and integrity is paramount. The potential for bias in AI models must be carefully addressed and mitigated through appropriate validation procedures. Data privacy and security concerns must be rigorously addressed to protect sensitive information. The computational resources required for running complex AI models can be substantial, potentially requiring upgrades to the data center infrastructure.
Comparison of AI Models for Scientific Data Analysis
Various AI models are suitable for scientific data analysis, each with its strengths and weaknesses. Deep learning models, with their ability to learn complex patterns from large datasets, are well-suited for tasks such as image recognition and prediction. Machine learning models, particularly those based on supervised learning, are effective for tasks requiring explicit labeling of data, such as classification and regression.
Statistical models, including Bayesian networks and Hidden Markov Models, are often preferred for tasks involving probabilistic reasoning and time-series analysis. The choice of model depends on the specific scientific problem and the nature of the data being analyzed.
Overview of the Coreweave AI Platform
Coreweave AI is a comprehensive platform designed for high-performance AI applications. Its key features include a scalable architecture that can handle massive datasets and complex algorithms. Advanced tools for model training and deployment are provided, streamlining the process of integrating AI into workflows. The platform integrates seamlessly with existing data infrastructure, minimizing disruption to ongoing research activities.
This integration facilitates efficient data exchange and processing between the platform and the data center’s resources.
Data Formats Supported by Coreweave AI and the Core Scientific Data Center
The seamless integration of Coreweave AI with the core scientific data center depends on compatible data formats. This compatibility ensures the efficient flow of data between the platform and the data center’s storage and processing systems.
Data Format | Coreweave AI Compatibility | Core Scientific Data Center Support |
---|---|---|
CSV | Yes | Yes |
JSON | Yes | Yes |
Parquet | Yes | Yes |
NetCDF | Yes | Yes |
HDF5 | Yes | Yes |
SQL databases | Potentially, depending on implementation | Yes |
Scalability and Capacity
A core scientific data center housing 1-2 billion Coreweave AI cores demands unparalleled scalability and capacity. This level of compute power necessitates sophisticated architectural designs and robust hardware/software solutions to ensure high throughput, low latency, and efficient data management. The core challenge lies in maintaining performance and reliability while handling massive datasets and complex computations.
Scalability Requirements
The sheer volume of data generated by such a large-scale AI system necessitates a highly scalable infrastructure. Scalability is critical to accommodate future growth and adapt to evolving scientific needs. This includes the ability to dynamically add or remove computing resources as required, ensuring optimal performance at all times. The architecture must anticipate the increasing complexity of scientific models and algorithms, allowing for future upgrades and expansions without significant disruption.
High Throughput and Low Latency
Achieving high throughput and low latency is paramount for real-time data processing and analysis. A distributed architecture, employing multiple interconnected nodes, can distribute workloads effectively, minimizing latency. Implementing caching mechanisms at various levels can significantly reduce the time required to access frequently used data. Furthermore, specialized hardware accelerators, such as GPUs and FPGAs, can be integrated into the system to accelerate specific computations.
Architectural Designs for Data Management
The data center’s architecture must support efficient data storage, retrieval, and processing. A tiered storage system, combining high-capacity, cost-effective storage with high-performance SSDs for frequently accessed data, is a common approach. Data replication across multiple locations ensures data redundancy and disaster recovery. Data partitioning and indexing strategies optimize data retrieval speed and reduce query complexity. Advanced data compression techniques minimize storage requirements while maintaining data integrity.
Hardware and Software Solutions
Several hardware and software solutions can be utilized to manage massive datasets. High-performance computing clusters, using commodity servers connected by high-speed networks, can provide substantial computational power. Specialized storage systems, designed for massive datasets, are essential for efficient data management. Software solutions, such as Hadoop and Spark, provide frameworks for distributed data processing and analysis. Cloud-based solutions offer scalability and flexibility, enabling dynamic resource allocation based on demand.
The Core Scientific Data Center 1, boasting 2 billion Coreweave AI processing power, is impressive. However, it’s crucial to consider the implications for organized crime, like those highlighted in the recent Europol AI Crypto Organized Crime Threat Report europol ai crypto organized crime threat report. This report shows how advanced AI can be weaponized, meaning the sheer power of the Core Scientific Data Center 1 also needs to be carefully managed to prevent misuse.
This massive computational capacity, while useful for many purposes, demands proactive measures to safeguard against potential criminal exploitation.
Data Storage Solutions
- HDD (Hard Disk Drives): HDDs offer high capacity at a lower cost, suitable for archival storage and less frequently accessed data. Their lower cost per gigabyte makes them ideal for long-term storage. Their relatively high latency makes them unsuitable for high-throughput, low-latency tasks.
- SSD (Solid State Drives): SSDs provide significantly faster read/write speeds compared to HDDs, making them ideal for frequently accessed data. Their high cost per gigabyte makes them better suited for caching and frequently accessed data. SSD storage systems are well-suited for workloads requiring fast response times.
- Cloud Storage: Cloud storage solutions offer scalability and flexibility, enabling dynamic resource allocation based on demand. They provide redundancy and disaster recovery options, making them suitable for storing critical data.
Data Storage Solution | Suitability for Core Scientific Data Center |
---|---|
HDD | Archival storage, infrequently accessed data |
SSD | Frequently accessed data, caching |
Cloud Storage | Scalability, flexibility, redundancy |
Data Management and Processing
The core scientific data center’s success hinges on its ability to efficiently manage and process the vast amounts of data generated by experiments and observations. Robust data management pipelines are crucial for ensuring data quality, facilitating analysis, and ultimately enabling scientific breakthroughs. The coreweave AI integration plays a pivotal role in optimizing these processes, accelerating insights, and reducing human error.
Data Ingestion, Validation, and Quality Control
The data ingestion process in the core scientific data center is meticulously designed to handle diverse data formats and volumes. Data sources range from high-throughput instruments to remote sensors, necessitating flexible ingestion protocols. Validation procedures are implemented to ensure data integrity. These procedures include checks for inconsistencies, missing values, and outliers, enabling a high degree of data quality control.
A critical aspect of this stage is the early identification and correction of errors, preventing downstream issues. Data validation is performed using standardized algorithms and pre-defined thresholds, minimizing the risk of erroneous results.
Data Transformation and Standardization
Data transformation and standardization procedures are essential to ensure compatibility across different data sources and analysis tools. This involves converting data into a unified format, such as a common data model. Standardization processes include normalizing units, handling missing values, and converting data types. The coreweave AI system facilitates the automation of these transformations, ensuring accuracy and consistency.
Data standardization allows for seamless integration with various analysis tools, maximizing efficiency and reducing errors.
Data Analysis and Interpretation
Analyzing and interpreting scientific data requires sophisticated tools and techniques. Modern methods encompass statistical analysis, machine learning algorithms, and visualization tools to extract meaningful insights from the data. The coreweave AI system provides access to a vast library of pre-trained models and algorithms for analyzing complex datasets. Interpreting scientific data requires a thorough understanding of the context, experimental design, and potential biases.
Experienced scientists and data analysts, working alongside the AI system, interpret the results and draw conclusions based on established scientific principles.
Data Pipelines and Workflows
Efficient data pipelines and workflows are crucial for managing the massive datasets generated in scientific research. These pipelines are customized to accommodate specific experimental protocols and analysis needs. One example is a pipeline designed for processing genomic sequencing data. It involves data ingestion from sequencing instruments, quality control, alignment to reference genomes, and variant calling. Another example involves analyzing satellite imagery data for climate modeling.
This pipeline includes image preprocessing, feature extraction, and integration with climate models. These optimized pipelines streamline the entire data processing workflow, ensuring timely and accurate results.
Data Analysis Tools
A wide range of tools are used for analyzing and interpreting scientific data. The selection of the appropriate tools depends on the specific analysis needs and the nature of the data.
Tool | Functionality |
---|---|
Python (with libraries like Pandas, NumPy, Scikit-learn) | General-purpose programming language for data manipulation, analysis, and visualization. |
R | Statistical computing language and environment with extensive libraries for statistical analysis and visualization. |
MATLAB | Software environment for numerical computation, visualization, and algorithm development, particularly useful for signal processing and image analysis. |
SPSS | Statistical software package for data analysis, including descriptive statistics, hypothesis testing, and regression analysis. |
Tableau | Data visualization tool for creating interactive dashboards and reports. |
Security and Privacy
Protecting sensitive scientific data within a massive core scientific data center is paramount. The sheer volume and critical nature of the information necessitate robust security measures, encompassing encryption, access controls, and adherence to strict privacy regulations. This section details the essential security protocols and standards required to safeguard the integrity and confidentiality of the data housed in this cutting-edge facility.
Security Measures for Sensitive Data
Data security in a high-performance computing environment requires a multi-layered approach. Physical security measures, such as controlled access to the data center and environmental monitoring, are crucial. This includes robust surveillance systems, restricted access zones, and redundant power and cooling systems to minimize downtime and data loss due to hardware failures.
Privacy Considerations and Compliance Requirements
Privacy regulations, such as GDPR and HIPAA, dictate how personal information and sensitive data must be handled. Data centers handling scientific data must adhere to these regulations. This necessitates meticulous record-keeping of data access, usage, and storage locations, along with transparent data handling policies communicated to all authorized personnel.
Data Encryption and Access Control Mechanisms
Data encryption is fundamental to securing sensitive data. Strong encryption algorithms, like AES-256, are vital for protecting data at rest and in transit. Access control mechanisms, including multi-factor authentication and role-based access control, are equally critical to limiting unauthorized access. This approach ensures that only authorized personnel can access specific data subsets, based on their defined roles and responsibilities.
Security Protocols and Standards
Various security protocols and standards are essential for ensuring data integrity and confidentiality. These include, but are not limited to, the following:
- ISO 27001: This international standard provides a comprehensive framework for information security management systems. Implementing ISO 27001 ensures a structured approach to risk assessment, control implementation, and ongoing monitoring. This standard helps organizations like this data center meet regulatory requirements and industry best practices.
- NIST Cybersecurity Framework: This framework, developed by the National Institute of Standards and Technology (NIST), offers a set of guidelines and standards for organizations to improve their cybersecurity posture. It helps organizations identify, assess, and manage cybersecurity risks across the entire data lifecycle. This framework provides a comprehensive approach to enhance the security posture of the data center.
- Security Information and Event Management (SIEM): SIEM systems collect and analyze security logs from various sources to detect and respond to security incidents. In a large-scale data center, SIEM systems are critical for proactively identifying and responding to potential security threats.
Security Measures Effectiveness Table
Security Measure | Description | Effectiveness |
---|---|---|
Firewall | Network security system that monitors and controls incoming and outgoing network traffic | High, prevents unauthorized access to the network |
Intrusion Detection System (IDS) | Monitors network traffic for malicious activity | High, detects and alerts on potential threats |
Data Encryption | Transforms data into an unreadable format | High, protects data even if compromised |
Multi-factor Authentication | Requires multiple forms of authentication | High, adds an extra layer of security |
Access Control Lists | Defines who can access specific resources | High, limits access to authorized personnel |
Future Trends and Innovations
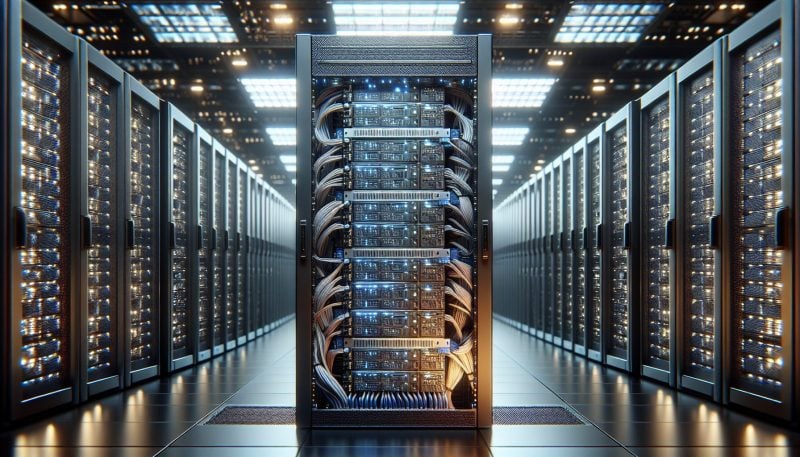
The future of scientific data centers hinges on embracing emerging technologies and adapting to the ever-increasing volume and complexity of scientific data. This requires proactive planning and investment in innovative solutions for storage, processing, and analysis. The integration of cutting-edge technologies like quantum computing and advanced AI will be crucial for unlocking new scientific discoveries and pushing the boundaries of human knowledge.
Emerging Technologies and Trends
The scientific landscape is constantly evolving, with new technologies continuously shaping the way data is managed and analyzed. Cloud computing, distributed ledger technologies (DLTs), and edge computing are becoming increasingly important in the context of scientific data centers. These advancements promise improved scalability, reduced latency, and enhanced data security. The rise of open-source software and collaborative platforms further facilitates data sharing and accelerates scientific progress.
Potential Future Developments in Data Storage
Future data storage solutions will likely leverage advanced storage technologies, such as holographic data storage, which offers the potential for significantly higher storage densities. 3D data storage is another area of active research, potentially providing massive capacity and faster access speeds compared to traditional methods. The use of novel materials and nanotechnologies for data storage is also expected to emerge, opening up the possibility of storing vast quantities of information in compact and energy-efficient ways.
Potential Future Developments in Data Processing
The evolution of data processing is moving towards more specialized and efficient architectures. Specialized hardware accelerators, tailored for specific scientific workloads, will become more prevalent. This will optimize processing speeds and reduce the computational resources needed to analyze massive datasets. Furthermore, the development of advanced algorithms for big data analysis will play a critical role in extracting meaningful insights from complex datasets.
Potential Future Developments in Data Analysis
The ability to analyze massive datasets will be further enhanced by the advancements in AI. Deep learning techniques will be crucial for uncovering patterns and relationships hidden within large datasets, leading to breakthroughs in various scientific disciplines. Moreover, the integration of AI into data analysis workflows will enable automation, accelerating the discovery process and reducing human error. Sophisticated visualization tools will be essential to effectively communicate the insights gained from complex analyses.
Impact of Quantum Computing and AI
Quantum computing promises to revolutionize scientific data management by enabling the solution of computationally intractable problems. Its ability to simulate complex molecular interactions and analyze vast datasets at unprecedented speeds will have a profound impact on scientific discovery. AI, in conjunction with quantum computing, will further enhance the capability to identify complex patterns and relationships within massive datasets, driving breakthroughs in fields like materials science, drug discovery, and astrophysics.
This combined approach is expected to significantly accelerate the pace of scientific advancement.
Innovative Approaches for Handling Massive Datasets
Handling massive datasets requires innovative approaches. Decentralized data storage systems, leveraging blockchain technology, can ensure data integrity and security, and distributed computing architectures can enable the parallel processing of large datasets across multiple servers. Furthermore, advanced data compression techniques will play a critical role in reducing storage requirements and improving processing speeds. This approach is expected to be more robust and reliable than traditional methods, which struggle to manage the increasing scale and complexity of scientific data.
Future Research Directions and Opportunities
The following areas present exciting opportunities for future research and development in the field of scientific data centers:
- Developing novel algorithms for processing and analyzing massive datasets in diverse scientific domains.
- Creating secure and scalable data storage solutions using advanced materials and technologies.
- Exploring the potential of quantum computing for accelerating scientific discovery and data analysis.
- Integrating AI into scientific workflows for automating data analysis and accelerating research.
- Developing advanced visualization techniques for effectively communicating insights from complex datasets.
Final Thoughts
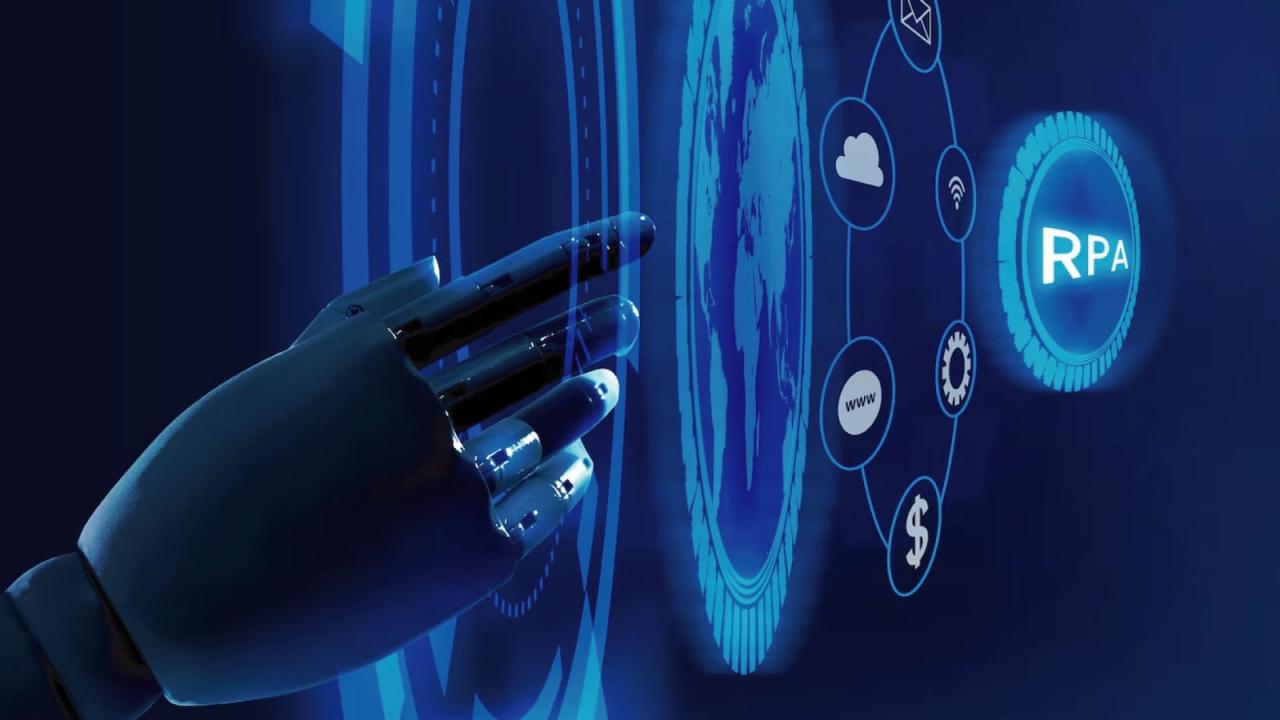
In conclusion, a core scientific data center of this scale, powered by Coreweave AI, represents a significant leap forward in scientific research. The ability to handle massive datasets and extract meaningful insights will undoubtedly revolutionize various fields. While challenges remain, the potential rewards are immense. We’ve explored the technical aspects, from infrastructure to data management, and highlighted the future potential for breakthroughs.
The future of scientific discovery is undeniably linked to the evolution of these advanced data centers.