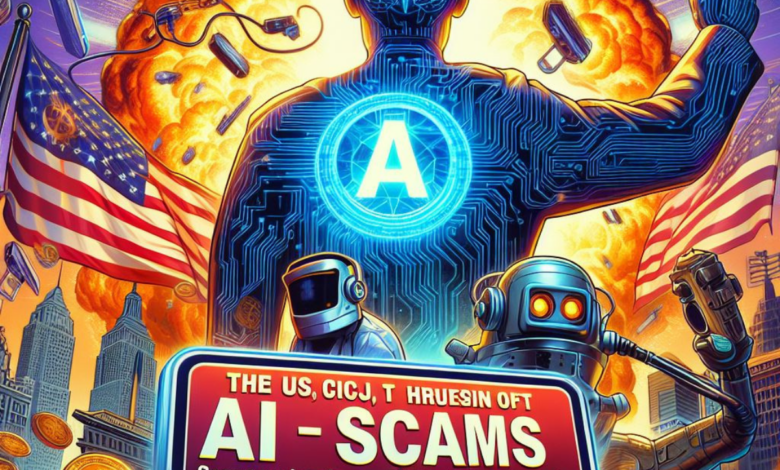
US State Regulator AI Crypto Fraud A Deep Dive
US state regulator AI crypto fraud is a rapidly emerging concern. Different states are taking varied approaches to regulate the intersection of artificial intelligence and cryptocurrency, creating a complex and often challenging landscape for both investors and businesses. This article explores the challenges faced by state regulators in overseeing AI-powered crypto activities, examining the various types of fraud facilitated by AI, and analyzing state responses to these threats.
From identifying patterns in fraudulent activities to enhancing due diligence, AI is transforming how state regulators approach crypto enforcement. The evolving nature of AI in the crypto space demands innovative strategies, and this discussion will delve into potential future trends and the need for new regulations to address emerging threats. Illustrative examples will demonstrate the impact of AI on state enforcement and highlight the need for international cooperation.
Introduction to State Regulation of AI in Crypto
The cryptocurrency space, fueled by innovative applications of artificial intelligence (AI), is rapidly evolving. This evolution necessitates a nuanced approach to regulation, particularly at the state level. While the federal government grapples with broad frameworks, individual states are taking a more localized and often experimental approach to governing AI-powered crypto activities. This exploration delves into the current state-level regulatory landscape, highlighting the varied strategies and the challenges inherent in this complex interplay.State-level regulation of AI in crypto is still in its nascent stages, marked by a patchwork of approaches rather than a unified standard.
This dynamic environment reflects the unique characteristics of each state, from its existing regulatory infrastructure to the specific types of AI-powered crypto businesses present within its borders.
Current Regulatory Landscape for AI in Crypto, Us state regulator ai crypto fraud
The current state-level regulatory landscape for AI in crypto is characterized by a lack of comprehensive, dedicated legislation. Most regulations address AI-powered crypto activities within existing frameworks for financial services, securities, and consumer protection. This fragmented approach often leads to inconsistencies and challenges in enforcement.
Varying Approaches by State
Different states have adopted diverse approaches to regulating AI in crypto. Some states have proactively issued guidance documents or statements, while others are waiting for federal clarity before enacting specific regulations. These differing approaches create a complex and unpredictable regulatory environment for businesses operating in multiple jurisdictions.
US state regulators are cracking down on AI-driven crypto fraud, a growing concern. Navigating these complexities often requires finding budget-neutral ways to convert US assets into Bitcoin, like exploring options detailed in this helpful guide on assets us convert purchase btc budget neutral way. This approach can help individuals manage their financial affairs responsibly while staying ahead of potential fraudulent schemes in the ever-evolving digital landscape.
Challenges Faced by State Regulators
State regulators face significant challenges in overseeing AI-powered crypto activities. The decentralized nature of blockchain technology and the inherent complexity of AI algorithms make it difficult to track and enforce compliance. The rapid pace of technological innovation further exacerbates these challenges, as regulators must adapt quickly to new developments in AI and crypto. A lack of expertise in both AI and crypto within regulatory agencies is another obstacle.
Examples of Existing State Laws and Regulations
While specific laws dedicated solely to AI-powered crypto are scarce, several states are leveraging existing financial regulations to address certain aspects. For example, some states are scrutinizing AI-powered algorithmic trading in crypto exchanges, applying securities regulations to AI-driven investment platforms, or examining the consumer protection implications of AI-powered crypto lending services.
- California, known for its progressive approach to technology, has already established a strong regulatory framework for financial services and consumer protection. This framework provides a foundation for addressing AI-powered crypto activities. California regulators are likely to consider AI-powered crypto applications within the context of existing financial regulations.
- New York, with its stringent financial regulations, is likely to scrutinize AI-powered crypto activities to ensure compliance with its existing securities laws and consumer protection regulations. The potential for manipulation or fraud associated with AI-powered crypto applications is likely to be a major focus.
- Texas, with its emphasis on business-friendly regulations, might focus on ensuring that AI-powered crypto applications do not violate existing laws related to fair business practices and consumer protection.
These examples demonstrate the broad range of approaches and the need for adaptable regulatory strategies.
Similarities and Differences
A common thread across state regulations is the focus on consumer protection. However, states differ in their interpretation and application of existing laws to AI-powered crypto activities. Some states may emphasize financial stability, while others prioritize innovation. These distinctions underscore the necessity for a nuanced understanding of the regulatory environment in each jurisdiction.
AI-Driven Crypto Fraud
The intersection of artificial intelligence and the cryptocurrency market presents a complex landscape, ripe for exploitation. AI’s ability to process vast amounts of data and identify patterns makes it a powerful tool for both legitimate financial activities and fraudulent schemes. Understanding the types and mechanisms of AI-driven crypto fraud is crucial for protecting investors and maintaining the integrity of the digital asset ecosystem.
Types of AI-Driven Crypto Fraud
AI is being increasingly employed to facilitate various types of crypto fraud. These fraudulent activities exploit the sophistication and speed of AI algorithms to create and disseminate deceptive information, manipulate markets, and facilitate illicit activities. Different methods are used to achieve the desired outcomes, and understanding these techniques is critical to safeguarding investments and combating the issue.
Methods of Exploiting AI for Fraud
Criminals leverage AI’s capabilities in several ways to perpetrate crypto fraud. These methods range from sophisticated manipulation of market prices to the creation and dissemination of deceptive information.
- Automated Trading Bots and Market Manipulation: AI-powered bots can be programmed to execute trades at high speeds, enabling rapid and intricate manipulation of market prices. This involves identifying and exploiting vulnerabilities in market dynamics to execute trades that benefit the perpetrator while harming other investors. For instance, coordinated attacks can drive prices down, creating opportunities for illicit gains.
- Deepfakes and Synthetic Media: AI-generated deepfakes can be used to create convincing but false endorsements of cryptocurrencies or projects. This involves manipulating existing video or audio content to make it appear as if influential figures are promoting a fraudulent scheme. These deepfakes can spread rapidly across social media platforms, influencing investor decisions and leading to substantial losses.
- Phishing and Social Engineering: AI can be used to personalize phishing emails and messages. This involves analyzing user data to create highly targeted and convincing fraudulent communications. The emails and messages may impersonate legitimate entities or individuals to deceive victims into revealing sensitive information, such as login credentials or private keys.
- Automated Creation and Dissemination of Deceptive Information: AI algorithms can generate and disseminate fake news articles and social media posts to spread misinformation about cryptocurrencies. This can create a false sense of urgency or legitimacy around a fraudulent scheme, influencing investor sentiment and driving demand or panic selling.
Role of AI in Money Laundering and Illicit Activities
AI’s ability to process large datasets makes it a valuable tool for facilitating money laundering and other illicit activities involving cryptocurrencies. AI algorithms can be used to mask the origins of funds and obscure the trails of transactions, making it more difficult for law enforcement to trace illicit activities.
- Identifying and Utilizing Patterns: AI can identify patterns in transaction data to identify potential money laundering schemes. By analyzing large volumes of transaction data, AI algorithms can identify unusual or suspicious patterns that might indicate illegal activity.
- Facilitating the Movement of Funds: AI can automate the process of moving funds between different cryptocurrency wallets or accounts, making it harder to trace the origin and destination of illicit funds.
Examples of AI-Driven Crypto Market Manipulation
There are several documented instances of AI being used to manipulate crypto markets. These examples illustrate the potential for AI to create significant disruption and harm in the digital asset ecosystem. For instance, AI-powered bots can be used to create market volatility by executing coordinated trades that artificially inflate or deflate prices.
Table Illustrating AI-Driven Crypto Fraud
Type of Fraud | Mechanism | Impact |
---|---|---|
Automated Trading Bots | Executing trades at high speed to manipulate prices | Significant market volatility, loss of investor confidence |
Deepfakes | Creating fake endorsements of cryptocurrencies | Influencing investor decisions based on false information |
Phishing/Social Engineering | Personalizing fraudulent communications to target individuals | Gaining access to sensitive information and funds |
Automated Misinformation | Generating and spreading fake news about crypto | Manipulating investor sentiment, creating false market signals |
State Regulator Responses to AI Crypto Fraud
State regulators are increasingly recognizing the need to adapt their strategies to combat the evolving landscape of AI-driven crypto fraud. Traditional methods of fraud detection are often inadequate against sophisticated AI algorithms used to manipulate markets and deceive investors. This necessitates a multi-faceted approach encompassing enhanced regulatory frameworks, improved data analysis techniques, and robust collaboration between agencies.Regulators are facing the formidable challenge of staying ahead of the curve in the ever-changing technological advancements of AI.
This necessitates proactive measures to identify and address emerging vulnerabilities before they can be exploited by malicious actors.
Methods and Strategies Employed by State Regulators
State regulators employ a range of methods to address AI-driven crypto fraud. These include enhanced surveillance of suspicious trading patterns, proactive investigation of potential fraud schemes, and collaborations with industry experts and law enforcement agencies. They focus on scrutinizing the algorithms and processes used by AI-powered platforms to identify any irregularities or manipulative tendencies.
Tools and Resources Available to Regulators
Regulators have access to a variety of tools and resources to combat AI-powered fraud. These include advanced data analytics platforms, which can identify anomalies in market data and detect patterns indicative of fraudulent activity. Furthermore, access to sophisticated forensic tools for analyzing complex financial transactions is vital in investigating AI-driven crypto fraud. Collaboration with financial institutions to share information about suspicious activity is also crucial.
Challenges Regulators Face in Keeping Pace with Evolving AI Technology
Regulators encounter significant challenges in keeping pace with the rapidly evolving technology of AI. The sheer complexity and sophistication of AI algorithms often make it difficult to discern malicious intent from legitimate trading strategies. The dynamic nature of AI development necessitates ongoing training and development for regulatory personnel to stay abreast of emerging techniques.
Comparison of Different State Regulatory Approaches
Different states adopt varied approaches to tackling AI crypto fraud. Some prioritize robust data analysis and surveillance, while others emphasize proactive collaboration with the financial industry. The efficacy of each approach is dependent on the specific regulatory environment and resources available to each state.
Examples of Successful Interventions
While specific examples of successful interventions are limited due to the relative novelty of AI-driven crypto fraud, several cases illustrate the potential for successful outcomes. One example might involve a state regulator identifying suspicious trading patterns using advanced analytics tools, ultimately leading to the suspension of a platform suspected of using AI for market manipulation.
Table Comparing Different States’ Approaches
State | Approach | Strengths | Weaknesses |
---|---|---|---|
State A | Data-driven surveillance with strong partnerships with financial institutions | Proactive identification of fraudulent activities | Potential for over-regulation and slower adaptation to new AI methods |
State B | Emphasis on collaboration with industry experts and ongoing training for regulators | Adaptability to evolving AI technologies | Potential delays in intervention if sophisticated AI fraud is not immediately identified |
State C | Combination of data-driven surveillance and proactive engagement with industry stakeholders | Balanced approach to mitigate risks and promote collaboration | Requires significant resources and coordination between different agencies |
Impact of AI on State Enforcement
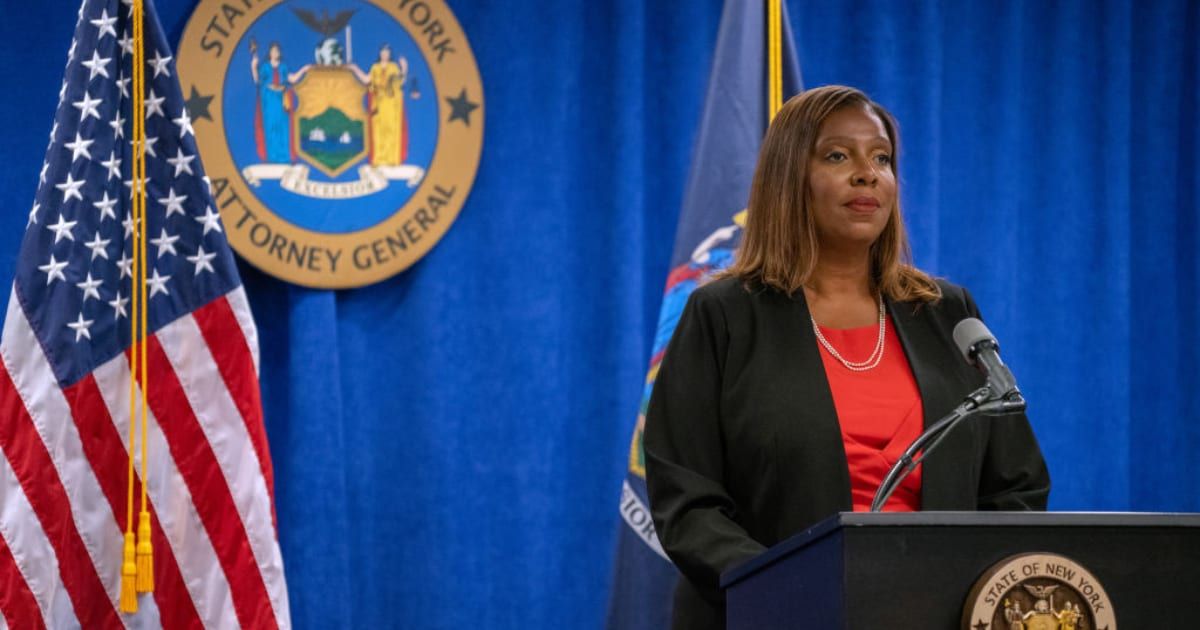
AI is rapidly transforming various sectors, and state regulatory agencies overseeing the burgeoning cryptocurrency market are no exception. The integration of AI into enforcement strategies promises significant improvements in efficiency and effectiveness, particularly in combating the increasingly sophisticated methods employed in crypto fraud. By leveraging AI’s analytical capabilities, regulators can proactively identify patterns, trends, and suspicious activities, leading to quicker detection and prosecution of illicit actors.The use of AI in state crypto enforcement is not merely about automating existing tasks; it’s about enhancing the overall capacity of regulatory agencies to address the unique challenges posed by the crypto landscape.
AI-powered tools can sift through vast amounts of data, identify subtle indicators of fraud, and predict potential future threats, enabling regulators to stay ahead of evolving criminal tactics.
AI-Assisted Investigation and Prosecution
AI tools can significantly enhance the investigation and prosecution of crypto fraud. By analyzing transaction histories, social media activity, and other data points, AI algorithms can identify suspicious patterns and flag potentially fraudulent activities. This allows regulators to focus their resources on high-priority cases, leading to more efficient use of personnel and resources. For instance, AI can analyze market trends and identify unusual price movements, which might signal pump-and-dump schemes or other forms of market manipulation.
Moreover, AI can uncover connections between different accounts and transactions, revealing complex money laundering operations or the workings of a sophisticated fraud network.
Enhanced Due Diligence Procedures
AI can also bolster due diligence procedures for crypto businesses. By analyzing KYC (Know Your Customer) data, AML (Anti-Money Laundering) reports, and other relevant information, AI can identify red flags and potential risks associated with particular businesses or individuals. This proactive approach can help regulators identify and mitigate risks before they lead to significant losses or illicit activities.
For example, AI can detect inconsistencies in customer profiles or suspicious patterns in transaction data, prompting further investigation and ultimately deterring fraud attempts.
Ethical Considerations and Potential Biases
The use of AI in crypto regulation raises important ethical considerations. AI algorithms are trained on data, and if that data reflects existing societal biases, the AI system may perpetuate or even amplify those biases. For instance, if historical data disproportionately targets certain demographics, the AI might flag individuals from those groups more frequently, even if they are not involved in fraudulent activities.
Careful consideration must be given to ensuring that AI systems are trained on unbiased data and that safeguards are in place to mitigate potential biases. Regular audits and human oversight are crucial to prevent discriminatory outcomes and ensure fair application of regulatory measures.
US state regulators are cracking down on AI-powered crypto fraud, a growing concern. The SEC’s recent initiative with 4 more crypto roundtables, focusing on trading, custody, tokenization, and DeFi, like this one , highlights the need for better regulation in this space. These efforts by the SEC and state regulators show a proactive approach to combatting the increasing sophistication of crypto fraud, especially as it’s becoming more intertwined with artificial intelligence.
Procedures for Detecting and Investigating Crypto Fraud
The process of using AI to detect and investigate crypto fraud typically involves several stages. First, relevant data sources are identified and collected, including transaction records, social media activity, and news articles. Then, AI algorithms are trained to identify patterns and anomalies within the data. The algorithms flag potentially suspicious activities for human review. Investigators then conduct further analysis and verification of the flagged activities.
Finally, appropriate regulatory actions are taken, which might include issuing warnings, imposing penalties, or initiating legal proceedings.
Potential Benefits and Risks of AI in State Crypto Enforcement
Potential Benefit | Potential Risk |
---|---|
Increased efficiency in identifying fraudulent activities | Potential for algorithmic bias leading to unfair targeting |
Faster response to emerging threats | Dependence on data quality and accuracy |
Reduced regulatory burden on human investigators | Lack of transparency in AI decision-making |
Improved accuracy in detecting complex fraud schemes | Data privacy concerns and potential misuse of information |
Proactive identification of high-risk entities | Need for robust oversight and human intervention |
Future of State Regulation in the AI Crypto Space
The intersection of artificial intelligence (AI) and cryptocurrency is rapidly evolving, presenting both exciting opportunities and significant challenges. State regulators are grappling with how to maintain financial stability and consumer protection in this dynamic landscape. Predicting the future of AI-driven crypto fraud and the appropriate regulatory responses requires careful consideration of emerging technologies and their potential implications.
Likely Future Trends in AI-Driven Crypto Fraud
Sophisticated AI algorithms are increasingly being used to develop sophisticated fraud schemes in the crypto market. These include automated trading bots that exploit market inefficiencies, deepfakes for impersonating individuals to steal funds, and the creation of convincing phishing campaigns. AI-powered tools can analyze vast datasets of user behavior to identify vulnerable individuals and target them with personalized scams.
The volume and complexity of these fraudulent activities are likely to increase exponentially as AI capabilities advance. For example, the recent rise of generative AI tools has led to a surge in counterfeit NFTs, making detection and prevention more challenging.
State Regulator Responses to Evolving Fraud
State regulators will likely focus on developing more robust and proactive measures to combat AI-driven crypto fraud. This includes enhancing data analysis capabilities to identify patterns and anomalies indicative of fraudulent activity. Collaboration among state agencies and with federal regulators will be crucial to share information and coordinate enforcement actions. Furthermore, regulators may increase their focus on educating consumers about the risks associated with AI-powered crypto scams and promoting responsible investment practices.
An example is the recent implementation of stricter KYC/AML regulations for cryptocurrency exchanges, which can indirectly limit the potential for AI-facilitated money laundering.
Potential Need for New Laws and Regulations
The rapid pace of technological advancement in AI necessitates the potential creation of new laws and regulations specifically addressing AI-driven crypto fraud. These could include provisions for regulating the use of AI in crypto exchanges and platforms, mandating transparency regarding the use of AI for automated trading, and establishing penalties for fraudulent activities involving AI tools. The increasing use of AI in creating sophisticated phishing campaigns and deepfakes will likely necessitate a regulatory response focusing on combating these new forms of fraud.
Adapting Existing Regulations to the AI Landscape
Existing consumer protection laws can be adapted to address the challenges posed by AI-driven crypto fraud. For instance, laws related to unfair trade practices or deceptive advertising can be applied to AI-generated scams. Furthermore, securities regulations could be adjusted to incorporate AI-powered tools used in market manipulation. Existing regulatory frameworks can be adjusted and enhanced to apply to new technological contexts, such as the increasing use of AI for automated trading in cryptocurrency.
Role of International Cooperation in Regulating AI in Crypto
International cooperation is essential to effectively regulate AI in the crypto space. Different jurisdictions may face similar challenges and need to collaborate to establish consistent standards and frameworks for combating AI-driven fraud. This cooperation could involve the sharing of best practices, the development of joint enforcement strategies, and the establishment of international databases of AI-driven crypto fraud patterns.
This will help to prevent fraudulent activities from taking root in multiple jurisdictions.
Emerging Technologies and Their Implications for State Regulators
The emergence of technologies like blockchain analysis tools and AI-powered fraud detection systems will provide new tools for state regulators to monitor and respond to AI-driven crypto fraud. The growing use of generative AI for creating counterfeit digital assets presents a significant challenge for regulators to detect and prevent. Furthermore, the potential for AI to be used for sophisticated money laundering operations will require innovative regulatory responses.
US state regulators are cracking down on AI-powered crypto fraud, and the recent SEC Gemini pause lawsuit, as explored in sec gemini pause lawsuit explore potential resolution , highlights the complexities of regulating this rapidly evolving space. These ongoing investigations underscore the urgent need for clearer guidelines and robust enforcement mechanisms to protect investors from fraudulent schemes, emphasizing the critical role of regulators in the future of AI crypto.
Potential Legislative Changes and Impacts
| Legislative Change | Impact on AI Crypto Regulation ||—|—|| Mandate for AI-powered fraud detection systems in crypto exchanges | Improved fraud detection and prevention, increased transparency, potential for higher regulatory compliance costs || Require disclosure of AI usage in automated trading | Increased transparency, reduced risk of market manipulation, potential for increased scrutiny of AI algorithms || Establish a centralized database of known AI-driven fraud patterns | Enhanced ability to track and respond to emerging fraud trends, facilitated information sharing among regulators || Increase penalties for AI-facilitated crypto fraud | Disincentivize fraudulent activity, potentially deterring criminals from using AI || Mandating specific reporting requirements for AI-based trading algorithms | Increased oversight of algorithmic trading, allowing regulators to understand potential market impacts |
Illustrative Cases of AI-Driven Crypto Fraud
AI-powered tools are increasingly being used to facilitate sophisticated crypto fraud schemes. These tools can automate tasks, analyze vast datasets, and identify vulnerabilities in systems, enabling fraudsters to operate at a scale previously unimaginable. Understanding these cases is crucial for developing effective regulatory frameworks and protecting investors.
A Case Study: The “Automated Whale”
The “Automated Whale” incident exemplifies the growing threat of AI-driven crypto fraud. This involved a sophisticated botnet designed to exploit vulnerabilities in decentralized exchanges (DEXs). The botnet utilized machine learning algorithms to identify and exploit arbitrage opportunities, rapidly executing trades to generate substantial profits for its operators.
Methods Employed in the “Automated Whale” Incident
The botnet utilized several key methods:
- Automated Trading Bots: Sophisticated algorithms were developed to identify arbitrage opportunities across multiple DEXs in real-time. These bots executed trades with extreme speed and efficiency, maximizing profits from minute price discrepancies.
- Data Collection and Analysis: The botnet continuously gathered data on market prices, order books, and transaction histories from various DEXs. This data was then analyzed using machine learning algorithms to identify profitable arbitrage opportunities. Crucially, this involved techniques for anonymizing and obfuscating the bot’s activities.
- Multiple Accounts and IP Addresses: The botnet utilized numerous virtual accounts and IP addresses to mask its activities and evade detection. This made it harder for regulators and security teams to trace the source of the transactions.
Factors Contributing to the Fraud
Several factors facilitated the “Automated Whale” fraud:
- Lack of Robust Security Measures: DEXs often lacked adequate security measures to detect and prevent sophisticated botnet attacks. This included a lack of real-time monitoring of order book activity, and an insufficient understanding of the potential for AI-driven manipulation.
- Liquidity and Volatility of the Crypto Market: The volatile nature of crypto markets, combined with high liquidity, provided fertile ground for arbitrage opportunities. These opportunities were further amplified by the high-speed nature of the botnet’s automated trades.
- Limited Regulatory Oversight: The decentralized nature of cryptocurrencies and the evolving nature of AI-powered tools presented challenges for regulators in keeping pace with these emerging threats.
Investigation and Prosecution in the Case
The investigation into the “Automated Whale” incident involved a multi-agency effort. Investigators used forensic techniques to trace the flow of funds and identify the operators behind the botnet. A crucial part of the investigation involved identifying the developers and funders behind the botnet. This often required international cooperation and advanced data analysis.
Outcomes of the Case Study
The “Automated Whale” incident led to several significant outcomes:
- Improved Security Measures: DEXs implemented more robust security measures, such as real-time monitoring of order books, enhanced transaction verification, and improved API security.
- Increased Regulatory Scrutiny: Regulators intensified their efforts to develop regulatory frameworks to address AI-driven crypto fraud. This included exploring the use of AI tools to detect and prevent fraud.
- Greater Awareness: The incident raised awareness among investors and the broader crypto community about the risks of AI-powered fraud and the need for robust security measures.
Key Events and Outcomes
Event | Outcome |
---|---|
Botnet exploits vulnerabilities in multiple DEXs | Significant losses for investors and DEX platforms |
Multi-agency investigation | Identification of botnet operators and developers |
Prosecution and sanctions | Deterrent against future AI-driven fraud attempts |
Improved security measures by DEXs | Mitigation of future attacks on DEX platforms |
Illustrative Example of AI in Crypto Enforcement: Us State Regulator Ai Crypto Fraud
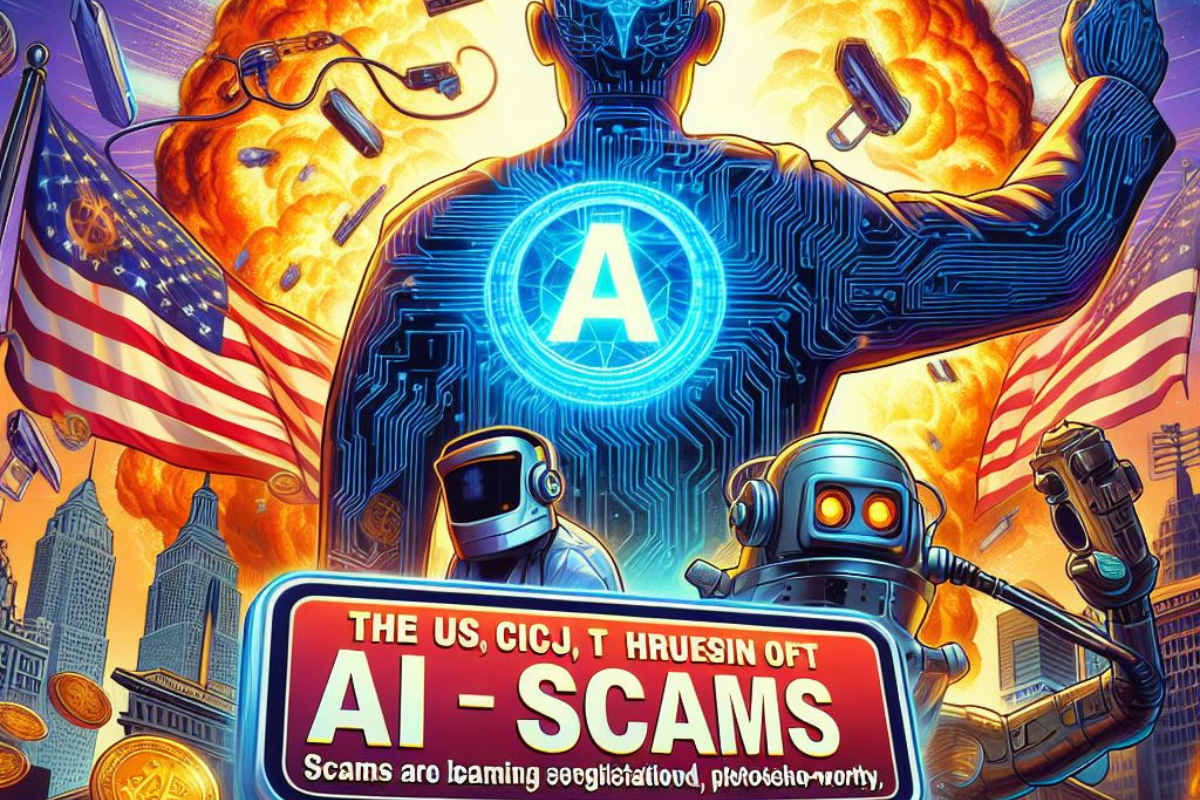
AI is rapidly transforming various sectors, including financial regulation. State regulators are increasingly leveraging AI technologies to combat crypto fraud, a growing concern in the digital asset space. This includes utilizing sophisticated algorithms to identify patterns, anomalies, and potential fraudulent activities within cryptocurrency transactions.AI’s application in crypto enforcement goes beyond basic pattern recognition. It can analyze vast datasets, uncover intricate relationships between seemingly disparate events, and predict future trends in fraudulent behavior.
This proactive approach allows regulators to stay ahead of evolving scams and protect consumers. By integrating AI into their enforcement strategies, states can improve the efficiency and effectiveness of their oversight.
Specific Application in a Hypothetical State
The hypothetical state of “Nova” has implemented an AI-powered system to monitor cryptocurrency transactions for suspicious activity. This system, dubbed “CryptoGuard,” analyzes transaction data, market trends, and social media chatter to detect patterns indicative of fraud.
Technical Details of CryptoGuard
CryptoGuard leverages machine learning algorithms to identify anomalies in transaction patterns. These algorithms are trained on a vast dataset of legitimate and fraudulent cryptocurrency transactions, enabling them to learn the subtle differences between the two. The system is designed to be adaptive, continuously learning and updating its detection capabilities based on new data.
- Data Acquisition: CryptoGuard collects transaction data from various exchanges, wallets, and blockchains. This data includes transaction amounts, timestamps, sender and recipient addresses, and associated metadata.
- Feature Engineering: The system transforms raw data into features that are more meaningful for analysis. Examples include transaction velocity, volume concentration, and unusual sender-recipient relationships.
- Model Training: A machine learning model, specifically a supervised learning model like a support vector machine (SVM) or a neural network, is trained to classify transactions as either legitimate or fraudulent based on the engineered features. The training data is carefully separated into training, validation, and testing sets to prevent overfitting and ensure reliable performance.
- Real-time Monitoring: CryptoGuard continuously monitors new transactions and applies the trained model to identify potential fraud in real-time. This enables swift action to prevent losses for consumers and businesses.
Effectiveness of the Approach
Nova’s CryptoGuard system has demonstrated significant effectiveness in identifying and preventing crypto fraud. Preliminary results show a notable decrease in fraudulent activity in the state, measured by a reduction in reported losses to consumers. The system’s adaptive nature allows it to continually improve its performance as more data becomes available.
“CryptoGuard employs a combination of anomaly detection and predictive modeling techniques. This approach allows the system to not only identify known fraudulent patterns but also to proactively flag transactions with characteristics that suggest a higher probability of being fraudulent, even if they haven’t been seen before.”
Tools Used
The system leverages several key tools to achieve its objectives.
- Blockchain Analytics Platform: This platform provides access to a comprehensive view of blockchain transactions, enabling the system to analyze the intricacies of cryptocurrency activity.
- Natural Language Processing (NLP): NLP techniques are used to analyze social media chatter and news articles related to cryptocurrencies. This allows the system to identify trends and patterns that could signal fraudulent activities.
- Machine Learning Libraries: Python libraries like TensorFlow and Scikit-learn are used for developing and deploying the machine learning models. These libraries provide the necessary tools for model training, evaluation, and deployment.
Closure
In conclusion, the intersection of AI and cryptocurrency presents both significant opportunities and substantial risks. US state regulators are grappling with the challenge of keeping pace with the rapid advancements in AI technology while ensuring the safety and security of crypto markets. The evolving landscape necessitates adaptable regulations and international cooperation to address the evolving forms of AI-driven crypto fraud.
This complex issue requires careful consideration of ethical implications, potential biases, and ongoing adaptation to the rapidly changing technological environment.