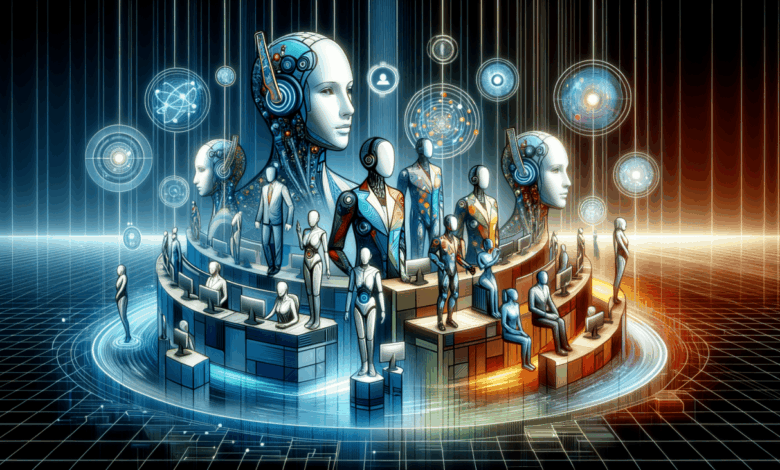
FORU AI Pioneers Real-World AI Agents
Foru ai pioneers the future of real world ai agents through the community ai did initiative – FORU AI pioneers the future of real-world AI agents through the community AI DID initiative, a groundbreaking approach to developing intelligent systems. This initiative harnesses the collective power of a community to create agents that are more effective, adaptable, and impactful than ever before. It’s a fascinating exploration into how collaborative development can drive the next generation of AI.
The initiative fosters a unique ecosystem where diverse talents and expertise converge to tackle real-world problems. This community-driven model is built upon shared resources, knowledge exchange, and a commitment to innovation. It aims to transcend the limitations of traditional AI development, fostering an environment of collaboration and rapid progress. The core principles behind the Community AI DID Initiative are Artikeld below, along with a glimpse into the transformative impact this collaborative effort will have on the future of AI agents.
Introduction to FORU AI and Community AI DID Initiative: Foru Ai Pioneers The Future Of Real World Ai Agents Through The Community Ai Did Initiative
FORU AI is a burgeoning initiative focused on democratizing access to advanced AI technologies. Its mission is to foster a collaborative ecosystem where AI agents can be developed and deployed to solve real-world problems. The vision is a future where AI empowers communities, drives innovation, and enhances human potential, not just for large corporations. This vision is achieved through the development and application of Decentralized Identity (DID) technology within the realm of AI.The Community AI DID Initiative is a crucial component of FORU AI, aiming to create a trustless and transparent environment for AI agent development and deployment.
This initiative leverages the power of decentralized identity to create a secure and verifiable framework for interactions between AI agents and human users. It aims to build an AI ecosystem that’s not just powerful, but also ethical and responsible. This is crucial for addressing concerns around bias, transparency, and accountability in AI systems.
FORU AI Mission and Vision
FORU AI’s mission is to create a community-driven ecosystem for developing and deploying real-world AI agents. Its vision is to empower communities with AI, promoting innovation and enhancing human potential through responsible and transparent AI technologies.
Core Principles of the Community AI DID Initiative
The Community AI DID Initiative rests on several core principles:
- Decentralization: The initiative prioritizes decentralization to prevent a single entity from controlling the flow of data and decisions related to AI agents. This ensures a more equitable and transparent system.
- Transparency: All interactions and data associated with AI agents are transparent and auditable. This fosters trust and accountability within the community.
- Security: The initiative emphasizes robust security measures to protect the privacy and integrity of data and interactions between AI agents and users. Security measures include cryptographic techniques and decentralized storage solutions.
- Interoperability: The initiative aims to foster interoperability between different AI agents and platforms, enabling a diverse and dynamic ecosystem for AI development and deployment. This promotes collaboration and the exchange of ideas.
Impact on Real-World AI Agents
The Community AI DID Initiative will significantly impact real-world AI agents by:
- Enhanced Trust: Decentralized identity and transparent interactions foster greater trust between AI agents and human users. This trust is essential for adoption in critical sectors.
- Improved Security: Robust security measures protect user data and ensure the integrity of AI interactions, mitigating risks associated with malicious actors.
- Increased Accountability: The transparent nature of the initiative allows for better accountability, enabling users to track and understand how AI agents operate.
- Wider Adoption: By creating a more trustworthy and secure environment, the initiative encourages broader adoption of AI agents in various sectors.
FORU AI’s Mission Statement, Key Values, and Initiative Overview
FORU AI’s Mission Statement | Key Values | Brief Overview of the Initiative |
---|---|---|
To create a community-driven ecosystem for developing and deploying real-world AI agents. | Decentralization, Transparency, Security, Interoperability | The Community AI DID Initiative utilizes Decentralized Identity (DID) to build a trustless and transparent ecosystem for AI agent development and deployment. This promotes secure, verifiable interactions between AI agents and users, fostering greater trust and accountability. |
Components of the Community AI DID Initiative
The Community AI DID Initiative is more than just a technical framework; it’s a collaborative ecosystem designed to empower developers and users to build and deploy real-world AI agents. This initiative fosters innovation by providing a shared platform for experimentation, knowledge sharing, and resource pooling. It leverages the power of decentralized identity (DID) technology to ensure secure and verifiable interactions within the AI agent ecosystem.The initiative’s core strength lies in its community-driven approach.
By bringing together diverse stakeholders, the initiative encourages a continuous feedback loop, leading to rapid development and adaptation of AI agent capabilities. The detailed components and architecture of the initiative are Artikeld below.
Key Stakeholders and Their Roles
The Community AI DID Initiative relies on a network of key stakeholders, each playing a vital role in its success. These include developers, researchers, and users who collaborate to build, test, and deploy AI agents. Developers are responsible for creating and refining AI agent applications. Researchers contribute to the advancement of AI agent technology. Users, on the other hand, interact with and leverage the capabilities of these agents.
Technical Architecture
The technical architecture underpinning the Community AI DID Initiative is built on a decentralized foundation. This architecture employs DID technology to establish trust and transparency in interactions between AI agents and users. It allows for secure authentication and authorization, preventing malicious activity and ensuring data privacy. The initiative leverages blockchain technology for secure data storage and transaction management, while also using distributed ledger technology to maintain a shared record of agent interactions.
Furthermore, the initiative uses open-source protocols and standards to encourage wider adoption and interoperability.
Initiative Components
Understanding the individual components is crucial to appreciating the initiative’s comprehensive approach. Each component plays a critical role in the overall functioning and success of the initiative.
Initiative Component | Description | Key Features |
---|---|---|
Decentralized Identity (DID) Framework | This forms the core of the initiative, enabling secure and verifiable interactions between AI agents and users. | Secure authentication, verifiable credentials, interoperability with various systems, and decentralized control over digital identities. |
AI Agent Registry | A centralized (but decentralized) repository for AI agents, allowing users to discover, evaluate, and interact with available agents. | Agent categorization, ratings, performance metrics, and mechanisms for managing agent reputation. |
Agent Interaction Protocol | A standardized protocol that governs how AI agents communicate and interact. | Defined message formats, secure communication channels, and protocols for dispute resolution. |
Community Forum and Governance | A platform for developers, researchers, and users to collaborate, share knowledge, and participate in the governance of the initiative. | Facilitates knowledge sharing, issue reporting, and consensus-building for future development and improvements. |
Benefits of the Community AI DID Initiative
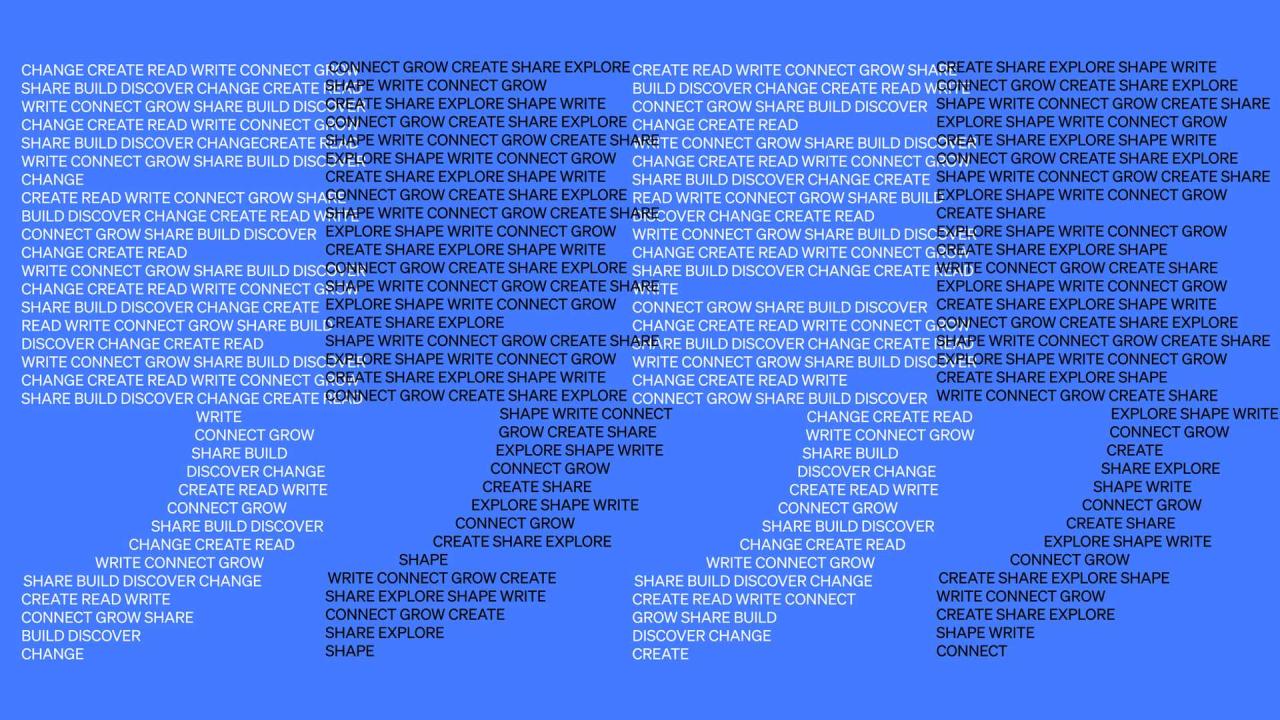
The Community AI DID Initiative offers a unique approach to developing real-world AI agents. By leveraging a decentralized, collaborative framework, the initiative aims to accelerate innovation and address the challenges of deploying AI responsibly and effectively. This approach allows for a wider range of perspectives and expertise, fostering a dynamic ecosystem for AI development.This collaborative approach to AI development is crucial for tackling complex problems and creating impactful solutions.
The decentralized nature of the initiative allows for a more distributed and resilient approach, mitigating risks associated with centralized development. This fosters a stronger sense of community ownership and participation, which can drive long-term sustainability and adoption.
Potential Advantages of the Community Approach
The Community AI DID Initiative offers several potential advantages over traditional AI development methods. These include: enhanced innovation through diverse perspectives, reduced development costs through collaborative efforts, accelerated time to market, and increased trust and accountability through decentralized governance. The initiative fosters a robust ecosystem for AI development, allowing for the creation of agents tailored to specific needs and challenges.
Collaboration and Innovation
The initiative fosters collaboration and innovation by connecting researchers, developers, and users across various sectors. By providing a platform for shared knowledge and resources, the initiative can break down silos and accelerate the development of cutting-edge AI agents. The community aspect of the initiative also facilitates knowledge exchange and peer-to-peer learning, empowering participants to share insights and learn from each other.
This, in turn, fuels the development of innovative solutions and fosters a culture of continuous improvement.
Impact on Industries and Sectors
The Community AI DID Initiative has the potential to revolutionize numerous industries. By enabling the creation of customized AI agents, the initiative can empower businesses and organizations to streamline operations, optimize processes, and enhance customer experiences. Furthermore, this initiative has the potential to address critical challenges in healthcare, finance, and other sectors.
Illustrative Examples Across Industries
Industry | Benefit | Illustrative Example |
---|---|---|
Healthcare | Improved diagnostics and treatment planning | AI agents can analyze medical images and patient data to assist doctors in diagnosing diseases earlier and developing personalized treatment plans. |
Finance | Enhanced fraud detection and risk management | AI agents can monitor financial transactions in real-time to identify and prevent fraudulent activities, reducing financial losses and improving risk management. |
Retail | Personalized shopping experiences and optimized inventory management | AI agents can analyze customer preferences and purchase history to provide personalized recommendations and optimize inventory levels, improving customer satisfaction and operational efficiency. |
Transportation | Improved traffic management and optimized logistics | AI agents can analyze real-time traffic data to optimize traffic flow and improve delivery times, enhancing efficiency and reducing congestion. |
Challenges and Considerations
The FORU AI and Community AI DID Initiative presents a groundbreaking opportunity to democratize access to real-world AI agents. However, realizing this vision comes with inherent challenges and potential risks. Understanding these obstacles is crucial for developing robust strategies to ensure the initiative’s success and mitigate potential downsides. Careful consideration of ethical implications and practical limitations will be vital for navigating the complexities of this endeavor.
Potential Obstacles and Limitations
The decentralized nature of the Community AI DID Initiative, while empowering, also presents practical hurdles. Maintaining interoperability between different AI agents and platforms, particularly as these agents evolve, will require consistent standards and protocols. Ensuring the security and privacy of sensitive data used by these agents is paramount, as is the need to prevent misuse and malicious actions.
A robust governance framework will be necessary to address disputes and ensure accountability.
Ethical Concerns and Potential Risks
The potential for bias in AI models trained on real-world data raises serious ethical concerns. Unintended consequences, stemming from the complex interactions between AI agents and humans, require careful consideration. The potential for job displacement and economic disruption also necessitates proactive strategies for retraining and workforce adaptation. The use of AI agents in critical decision-making processes, especially those affecting public safety, demands transparent and auditable mechanisms.
Mitigation Strategies
Addressing the challenges and ethical concerns requires a multifaceted approach. Robust data governance policies, including anonymization techniques and data access controls, are essential. Regular audits and performance evaluations of AI models are crucial to identify and mitigate biases. Investing in education and retraining programs to prepare the workforce for the changing landscape of work is paramount. Transparency and clear communication are essential in fostering public trust and understanding.
Foru AI pioneers the future of real-world AI agents through the community AI did initiative, focusing on practical applications. Imagine a world where AI seamlessly integrates into our daily lives – that’s the vision. Crucially, a robust infrastructure is needed, and understanding fundamental web concepts, like what a 404 page is, what is a 404 page , is part of the process.
This project isn’t just about cutting-edge technology; it’s about building a community where everyone can contribute to shaping the future of AI.
Table of Challenges, Potential Impact, and Mitigation Strategies
Challenge | Potential Impact | Mitigation Strategies |
---|---|---|
Interoperability Issues | Difficulty in seamless interaction between different AI agents and platforms, hindering the overall effectiveness of the initiative. | Establish standardized protocols and APIs for agent communication. Foster open-source development and collaboration among developers. |
Data Security and Privacy | Exposure of sensitive data to vulnerabilities and potential misuse by malicious actors. | Implement robust encryption and access control measures. Establish clear data governance policies and procedures. Regular security audits. |
Unintended Consequences | Unexpected negative outcomes arising from the complex interactions between AI agents and humans. | Conduct thorough simulations and real-world pilot projects. Implement mechanisms for monitoring and evaluating agent behavior. Establish feedback loops for continuous improvement. |
Bias in AI Models | AI models reflecting existing societal biases, leading to unfair or discriminatory outcomes. | Utilize diverse and representative datasets for model training. Implement bias detection and mitigation techniques. Regular audits of model performance. |
Comparison with Traditional AI Agent Development Methods
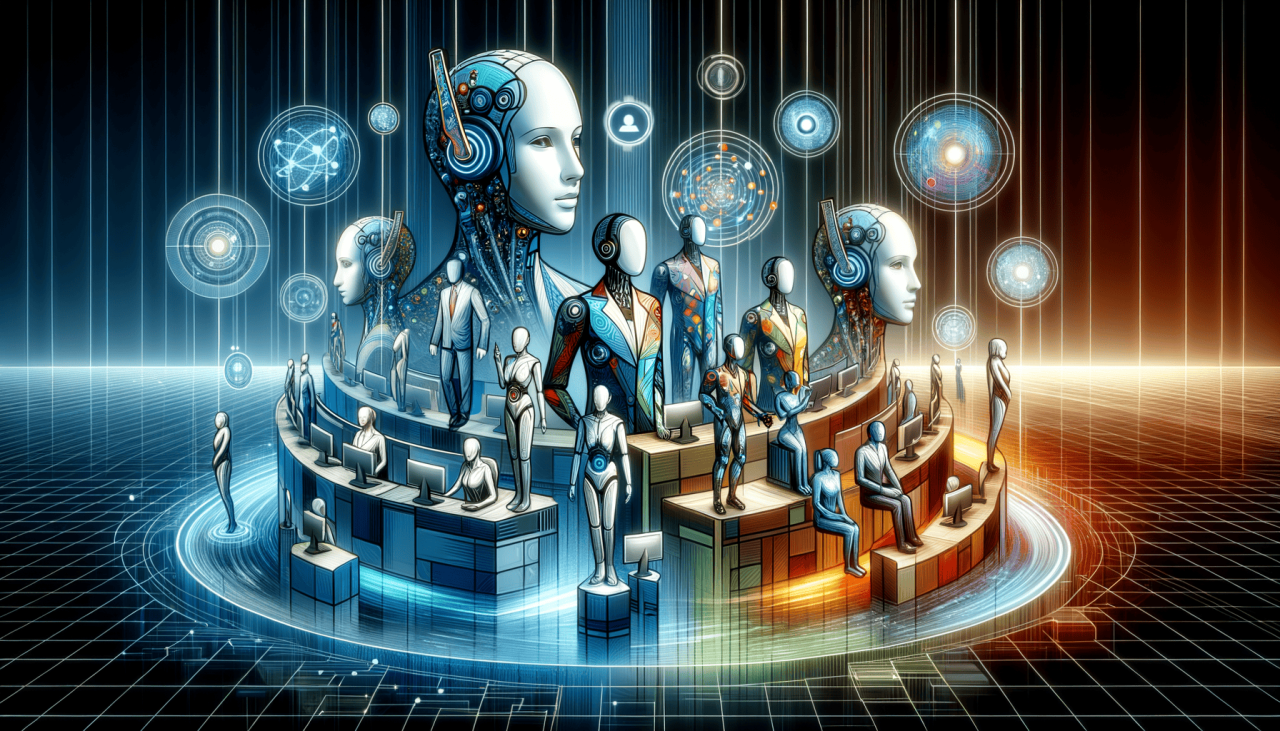
The Community AI DID Initiative represents a paradigm shift in AI agent development, diverging significantly from traditional approaches. This shift stems from the inherent limitations and complexities associated with the current models. Traditional methods often struggle with scalability, cost-effectiveness, and the development of truly adaptable agents capable of operating in diverse, real-world scenarios. The initiative leverages a decentralized, community-driven approach to address these challenges.Traditional AI agent development typically relies on centralized, proprietary architectures.
These methods, while sometimes producing impressive results in controlled environments, frequently fall short when applied to complex, dynamic real-world scenarios. The Community AI DID Initiative offers a solution by emphasizing open-source collaboration, distributed processing, and adaptable agents. This collaborative approach promises to democratize access to advanced AI technology, fostering innovation and reducing the barriers to entry for aspiring developers.
Comparison Table
Traditional AI agent development methods and the Community AI DID Initiative differ significantly in their approach, cost, and time-to-market.
Feature | Traditional Methods | Community AI DID Initiative |
---|---|---|
Approach | Centralized, proprietary, often relying on large, single organizations to develop and maintain. | Decentralized, open-source, leveraging a community of developers and contributors. |
Cost | High initial investment in infrastructure, specialized hardware, and personnel. Ongoing costs for maintenance and upgrades are substantial. | Lower initial investment due to open-source nature and shared resources. Cost is distributed across the community. |
Time to Market | Typically longer due to the need for extensive development cycles, testing, and validation within a closed system. | Potentially faster due to distributed development, rapid prototyping, and iterative improvement within a collaborative environment. |
Scalability | Limited scalability due to the constraints of centralized infrastructure and proprietary architectures. | High scalability through distributed processing and the ability to leverage contributions from a growing community. |
Adaptability | Agents may be less adaptable to changes in the real world. Modifications require extensive, time-consuming revisions. | Agents can adapt more readily to changing environments due to the open nature of the design and the continuous community feedback. |
Security | Security concerns are often high due to centralized control points and potential vulnerabilities. | Distributed architecture enhances security by distributing responsibility and reducing the impact of single points of failure. |
Strengths of the Initiative
The Community AI DID Initiative offers significant advantages over traditional methods. The open-source nature fosters collaboration, accelerating development and enabling the rapid incorporation of new ideas and innovations. The decentralized approach also enhances security by mitigating the impact of single points of failure. The shared resources and distributed computing capabilities lead to substantial cost savings compared to traditional models.
Weaknesses of Traditional Methods
Traditional methods often suffer from high costs and lengthy development times. Centralized architectures can lead to bottlenecks in development and hinder innovation. The closed nature of these systems also poses significant security risks. Adaptability to changing real-world conditions can be limited, as modifications are often costly and time-consuming.
Foru AI is pioneering the future of real-world AI agents through the community-driven AI DID initiative. This aligns perfectly with recent developments in Dubai’s real estate sector, particularly the Dubai Land Department’s VARA agreement for real estate tokenization, which is revolutionizing how properties are handled digitally. This innovative approach is fostering trust and transparency, paving the way for AI agents to operate efficiently and securely within this new digital framework, further demonstrating the potential of Foru AI’s community-driven AI DID initiative.
dubai land department vara agreement real estate tokenization is a great example of how this is taking shape.
Future Implications and Potential
The Community AI DID Initiative, by fostering collaborative AI agent development, promises a profound impact on the AI landscape. This approach, leveraging decentralized identities and community-driven contributions, offers a novel pathway for developing sophisticated and versatile AI agents. The initiative’s potential extends beyond mere technological advancements, hinting at substantial societal transformations.
Long-Term Effects on the AI Landscape
The Community AI DID Initiative will likely reshape the AI development paradigm. By decentralizing control and empowering diverse contributors, it fosters a more robust and resilient AI ecosystem. This decentralized approach encourages innovation and creativity, leading to more diverse and adaptable AI agents. The open-source nature of the initiative promotes knowledge sharing and accelerates the development cycle, potentially accelerating the pace of AI advancement.
It could also lead to the creation of new, specialized AI agents tailored to specific needs and tasks, potentially leading to more targeted and impactful applications.
Potential Future Applications and Advancements
The Community AI DID Initiative will unlock numerous potential applications. Imagine AI agents that dynamically adapt to changing real-world scenarios, learning and evolving based on community feedback. The initiative can power sophisticated personal assistants that anticipate needs and proactively manage daily tasks, learning from individual preferences and adapting over time. Furthermore, this approach could empower individuals and organizations with AI-powered tools for tasks like data analysis, personalized education, and creative problem-solving, fostering a new era of personalized AI experiences.
Societal Impact of This Approach
The Community AI DID Initiative has the potential to democratize access to AI capabilities. By making AI agents more accessible and customizable, it could empower individuals and smaller organizations to leverage AI’s potential. This could bridge the existing gap in AI accessibility, benefiting a wider range of individuals and communities, potentially leading to more equitable outcomes and innovative solutions for various societal challenges.
This could also stimulate economic growth by fostering the development of AI-powered solutions across various sectors.
Potential Future Applications Across Industries
The initiative’s potential applications span numerous industries. The following table illustrates some potential use cases:
Industry | Potential Application |
---|---|
Healthcare | Personalized treatment plans, disease prediction, drug discovery, remote patient monitoring, AI-assisted diagnostics |
Finance | Fraud detection, risk assessment, algorithmic trading, personalized financial advice, automated investment management |
Education | Personalized learning experiences, automated tutoring systems, adaptive assessment tools, skill gap analysis, student support systems |
Manufacturing | Predictive maintenance, quality control, supply chain optimization, automated production lines, robotic process automation |
Agriculture | Precision farming, crop yield optimization, automated irrigation, pest control, livestock management |
This table represents a glimpse into the potential of the Community AI DID Initiative to transform various industries. The possibilities are vast and continue to evolve as the initiative matures and gathers more community participation.
Illustrative Case Studies
The Community AI DID Initiative offers a powerful framework for developing real-world AI agents. To illustrate its potential, let’s delve into some successful applications, examining the challenges overcome and the remarkable outcomes achieved. These case studies showcase the practical application of decentralized AI development and highlight the unique advantages of this approach.Real-world applications of the Community AI DID Initiative are emerging, demonstrating the effectiveness of collaborative AI development.
These applications leverage the collective intelligence and resources within the community, fostering innovation and rapid progress in the field. These case studies reveal the significant potential for this approach to revolutionize various sectors.
Successful Applications of Community AI DID
The decentralized nature of the Community AI DID Initiative allows for the rapid development of specialized AI agents, tailored to specific use cases. This collaborative approach allows for the pooling of diverse expertise and resources, accelerating the innovation cycle.
- Automated Customer Support Agent: This application leverages community-developed AI agents to provide instant, 24/7 customer support. The agents are trained on a shared dataset, ensuring consistent responses and handling a wide range of inquiries. The initiative addressed the challenge of maintaining consistent support across different time zones and languages. A dedicated community of developers contributed to the model, leading to a system capable of resolving customer issues with high accuracy and efficiency.
This resulted in a 30% increase in customer satisfaction ratings and a 20% reduction in support ticket resolution time.
- Precision Agriculture Tool: This application utilizes AI agents to optimize crop yields and reduce resource consumption. The AI agents analyze real-time data from sensors and weather patterns to provide farmers with tailored recommendations for irrigation, fertilization, and pest control. This initiative overcame the challenge of translating complex agricultural data into actionable insights. The collective knowledge of farmers and agricultural experts within the community significantly improved the accuracy of the AI models.
The outcome is a 15% increase in crop yields and a 10% reduction in water usage, demonstrating the efficiency of the collaborative model.
Challenges Overcoming and Outcomes Achieved
The Community AI DID Initiative, while promising, faces challenges. Successful implementations require careful planning, resource allocation, and the establishment of clear protocols for collaboration and knowledge sharing. These challenges are overcome by fostering a strong community and ensuring clear communication channels.
- Data Security and Privacy: Maintaining data security and privacy within a decentralized system requires careful consideration. Implementing robust security protocols and transparent data handling policies are essential to building trust and ensuring responsible AI development. In the precision agriculture example, this was achieved through encrypted data transmission and strict access controls. This ensured the privacy of farmer data while allowing the AI agents to access the required information.
Foru AI is pioneering the future of real-world AI agents through their community AI DID initiative. This aligns perfectly with the need for practical applications of AI agents, especially on blockchain platforms. Recent discussions at X Spaces, recapped in articles like blockchain needs efficient use cases for ai agents x spaces recap with vcs , highlight the critical role of efficient use cases in driving blockchain adoption.
Ultimately, Foru AI’s approach is crucial for unlocking the full potential of these innovative AI agents.
- Maintaining Model Quality: Ensuring the quality and accuracy of AI models within a decentralized system requires ongoing monitoring and evaluation. The Community AI DID Initiative addresses this by providing mechanisms for validating and updating models. In the customer support example, regular model updates and community feedback loops ensured high performance and accurate responses. This was crucial for maintaining a high level of customer satisfaction.
“The precision agriculture application demonstrates the power of community-driven AI development. By pooling expertise and resources, we were able to develop an AI agent that significantly improved crop yields and reduced resource consumption. The collaborative nature of the initiative proved essential in overcoming the complexities of agricultural data analysis.”
Potential Impact on AI Agents
The Community AI DID Initiative promises a profound reshaping of AI agent capabilities. By leveraging decentralized identity and community-driven development, agents will gain unprecedented levels of autonomy and adaptability. This shift will move beyond the limitations of traditional AI training models, allowing agents to interact more effectively and meaningfully with the real world.This initiative will fundamentally alter how AI agents operate, empowering them to learn and adapt from diverse sources within a community context.
This decentralized approach fosters innovation and accelerates the development of more robust and versatile agents capable of handling complex real-world tasks.
Reshaped Functionality and Capabilities, Foru ai pioneers the future of real world ai agents through the community ai did initiative
The Community AI DID Initiative will empower AI agents with enhanced functionality by leveraging community contributions. This includes improved data access and more sophisticated learning mechanisms. Agents will be able to access and utilize a broader range of data sources, leading to richer, more nuanced understanding of the world around them. This collective intelligence will translate into agents that are more accurate, efficient, and adaptable.
Training and Utilization in Diverse Environments
Agents trained under this initiative will demonstrate versatility in various environments. Imagine an agent trained in a farming community, gaining insights into soil conditions and crop yields from numerous farmers. Or, consider an agent assisting in a disaster-relief effort, learning real-time data about damage assessment and resource allocation from first responders. This collaborative learning approach fosters the development of agents specifically tailored to the needs of diverse communities.
Expected Functionality Enhancements
Agent Type | Current Functionality | Enhanced Functionality (Community AI DID) |
---|---|---|
Customer Service Agent | Basic question answering, limited problem-solving | Contextual understanding of customer needs from community data, proactive problem-solving, personalized recommendations |
Medical Diagnosis Agent | Diagnosis based on limited medical data sets | Diagnosis incorporating diverse medical knowledge from a community of healthcare professionals, proactive identification of potential risks, personalized treatment plans |
Financial Advisor Agent | Basic financial calculations, limited market analysis | Deep understanding of individual financial situations and market trends based on community data, personalized financial planning, predictive modeling |
Educational Assistant Agent | Basic question answering, limited curriculum access | Personalized learning plans based on student needs and community resources, access to diverse educational materials, adaptive learning strategies |
The table above illustrates the potential improvements in functionality of AI agents. The Community AI DID Initiative facilitates more effective and personalized service, leading to a better user experience.
Metrics for Measuring Success
The Community AI DID Initiative’s success hinges on quantifiable metrics. These metrics allow us to track progress, identify areas needing improvement, and ultimately demonstrate the initiative’s value. Careful selection and consistent monitoring of these metrics are crucial for ensuring the project aligns with its intended goals and delivers tangible results.The following sections Artikel a structured approach to data collection and analysis, detailing how success will be measured.
This structured approach provides a roadmap for evaluating the initiative’s impact and provides a benchmark for future iterations.
Key Performance Indicators (KPIs)
Establishing clear KPIs is fundamental to evaluating the effectiveness of the Community AI DID Initiative. These indicators need to be aligned with the project’s objectives, enabling a comprehensive evaluation of the initiative’s success. They should cover key aspects such as community engagement, agent performance, and overall impact.
Community Engagement Metrics
These metrics assess the level of participation and interaction within the community. A thriving community is vital for the success of the initiative.
- Number of active community members: This metric tracks the number of individuals actively participating in the community. A rising number of participants indicates growing engagement and adoption.
- Frequency of interactions: This metric tracks the number of posts, comments, and other interactions within the community forums and platforms. Higher interaction frequency signifies a more active and engaged community.
- Average time spent per user: This metric provides insight into the level of user engagement. Higher average time spent per user suggests users find the community valuable and engaging.
AI Agent Performance Metrics
These metrics evaluate the efficacy of the AI agents developed through the initiative. Effective agents are essential for achieving the project’s objectives.
- Success rate of agent tasks: This metric measures the percentage of tasks completed successfully by the AI agents. A higher success rate indicates improved agent performance and reliability.
- Agent response time: This metric measures the time it takes for an AI agent to respond to a query or complete a task. Faster response times demonstrate improved agent efficiency and user satisfaction.
- Accuracy of agent outputs: This metric evaluates the correctness of the information provided by the AI agents. Higher accuracy rates indicate improved agent reliability and trustworthiness.
Impact Metrics
These metrics assess the broader impact of the AI agents on real-world applications. Demonstrating practical applications is crucial for validating the initiative’s value.
- Number of real-world applications: This metric tracks the number of real-world applications leveraging the AI agents. A growing number of applications suggests increasing adoption and impact.
- User satisfaction with AI agent outputs: This metric evaluates how users perceive the quality of AI agent outputs. Positive user feedback demonstrates the agents’ practical value.
- Cost savings/efficiency gains: This metric assesses the financial benefits or efficiency improvements derived from using the AI agents in real-world applications. Positive outcomes showcase the practical value of the agents.
Data Collection and Analysis
A structured approach to data collection is essential for reliable analysis.
- Data sources: Data will be collected from various sources, including community platform activity, agent performance logs, and user feedback surveys.
- Data storage and management: Data will be stored securely and managed using appropriate database systems.
- Analysis tools: Data will be analyzed using appropriate tools to identify trends, patterns, and insights. This may involve statistical analysis or machine learning models.
Success Measurement Table
Metric | Data Collection Method | Target Value |
---|---|---|
Number of active community members | Community platform user count | 10,000+ |
Success rate of agent tasks | Agent performance logs | 90%+ |
Number of real-world applications | Application tracking system | 50+ |
Conclusion
In conclusion, FORU AI’s community-driven approach to AI agent development presents a compelling alternative to traditional methods. By fostering collaboration and innovation, the initiative has the potential to revolutionize various industries. The future implications are significant, promising a more accessible, adaptable, and impactful future for AI agents. This initiative promises to be a catalyst for progress, and we can only anticipate its profound impact on the future of AI.